Engineering Antibodies In A Box?

By Matthew Pillar, Editor, Bioprocess Online
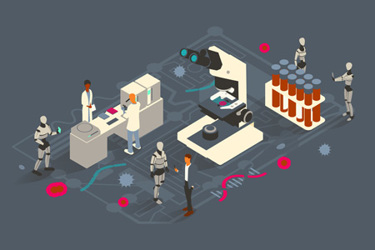
It's an anecdotal observation, but in my experience, scientists hold a healthy degree of skepticism toward the role of machine learning (ML) in biopharma drug discovery. That’s as it should be, given the nature of a business where next-level human scrutiny and interrogation are primary to the job.
On the other hand, there's a growing throng of startup biopharma companies to which ML is a cornerstone of discovery efforts. LabGenius is one such startup, and its founder and CEO is intent on marrying the best of the former – human skepticism and ingenuity – with the best of the latter – machine intelligence.
LabGenius is developing what it calls a smart robotic platform named Eva, which it claims capable of designing, conducting, and learning from its own experiments in an effort to discover new therapeutic antibodies. Importantly, it's applying that platform to the development of its own internal pipeline of therapeutic mono- and multi-specific antibody candidates in cancer and inflammatory diseases.
ML Garbage In, Garbage Out
Computational methods in the drug discovery process aren’t new, says Field, but he is observing a sea change in their adoption. “It’s now universally acknowledged that the deep integration of ML and computational methods into every stage of the drug discovery process is an inevitability, rather than maybe just a possibility,” he says. It’s an inevitability that, in his estimation, has seen hampered real-world results due to a lack of what he calls ML-grade data availability. In any data-driven enterprise, true data scientists know well and subscribe readily to the “garbage in, garbage out” adage. That old axe holds true—and with serious consequences—in biopharma. “For the majority of problems that drug hunters need to solve, the real big issue is there are no readily available data sets of the requisite quality for ML,” he says. “If you want to answer some of the most interesting and potentially impactful questions in drug discovery, then it means you actually have to generate new data and ensure from the outset that it's collected and structured with ML in mind.” The acknowledgment that ML is going to touch every stage of the drug discovery process will only come to fruition if skeptical and ingenious humans develop engines that can generate ML-grade data, an exercise Dr. Field calls far from trivial. “This requires a lot of integration, not only from the data generation and ML perspective, but also in terms of data capture, storage, and processing. I think that's the reason we're not further along in the process.”
That’s why much of the innovation we've seen in the early part of the ML adoption curve has been, for lack of a better term, proprietary. Some ML-intensive biopharma startups have jumped into the game with pre-existing, internal data sets or readily available, properly structured public data sets. Large, open data sets have proven valuable to answering questions about how proteins fold, for instance. But from Field’s perspective, the data doesn’t yet exist, at least, not in the right quality or quantity, to address some of the most interesting and fundamental questions drug hunters ask. Thus, the generation of data sets that specifically serve the drug discovery and design space is where we’ll see the most ML activity and growth in the short term.
Effects Of Protein Design On Molecular Performance
That dialed-in data set approach is exactly where LabGenius has zeroed its sights. The company is focused on antibody therapeutics, and more specifically, predicting and understanding how protein design impacts the way a molecule will perform in the context of specific disease cells. That’s very interesting to us, because historically, running those sorts of experiments is exceptionally low throughput. This is where we feel that ML has the biggest potential to provide an advantage in the discovery process.”
Even more specifically, LabGenius is applying its ML applications to the discovery and design of multi-specific, multivalent antibody therapeutics with complex mechanisms of action. It’s trying to understand how molecular attributes like geometry, valency, affinity, and topology impact performance. That’s all been done before, but LabGenius’ efforts depart from the norm in that the company is trying to optimize those attributes in parallel. Historically, protein engineers would engineer those attributes sequentially—one at a time—which is not only time consuming, but error-prone. “When you optimize one attribute, you often inadvertently de-optimize another,” says Field. By generating data for each feature of interest simultaneously, LabGenius can create computational models that are predictive of the performance impact of its engineering exercises, thus enabling the simultaneous optimization of multiple design attributes in one fell swoop, without violation of any Newtonian laws.
Bad Data Is A People Problem
Theoretically, the concept is elegant in its simplicity. But the real-world challenge Field admits to is the aforementioned “garbage-in, garbage-out” paradigm. To ensure the baseline data LabGenius is working with isn’t “garbage,” Field flies in the face of the misperception that current applications of ML are somehow fully autonomous. Instead, he presses the importance of tight integration between the company’s lab and computational scientists. “We’ve focused our innovation and engineering on ensuring the generation of the right data at the right quality and at the right throughput speed,” he says. “You might picture this technology stack that we're building like a pyramid. At the top, you have data analysis and ML. But the real foundation is the ability to generate, capture, store, and process data.” That baseline data requires very qualified human oversight and intervention that’s prerequisite to machine learning—ML simply won’t perform without it. “At each step, you must have really tight integration between the data generation domain experts and the ML domain experts,” says Field.
Importantly, facilitation of that integration is a two-way street that demands change from both the generators of lab data and the coders who feed the ML application its marching orders. “If you were to generate and capture data the conventional way a trained biologist might do it, you wouldn’t necessarily include all the quality controls that are essential for allowing the normalization and noise reduction required of a ML approach,” he explains. That requires some skillset realignment, but the mastery of this nontrivial integration, says Field, is LabGenius’ killer differentiator. Referencing the specific advantage of being a small, agile startup, he says big pharma, in particular, would struggle to re-engineer the processes necessary to achieve an effective marriage of the biological and computational domains. “When I started this company, I thought we were trying to solve an engineering problem, and maybe a computational problem, and maybe a scientific problem. But a major part of this is solving an organizational engineering challenge. And you know, I think being a startup gives us some nice advantage over the incumbents in the sense that, rather than having different teams that are siloed, in different parts of the world, you can get everybody in one room, and only through that really close and cross-functional collaboration can you really start bridging the divide between some of some of these different domains.”
If you want to learn about the fruit LabGenius’ efforts are bearing, you’ll have to tune in to episode 157 of the Business of Biotech podcast.