A Hybrid Modeling Approach To Accelerate Media And Feed Development
By Timo Schmidberger, Chloe Lang, Chris McCready, Frida Nordström, and Sinyee Yau-Rose
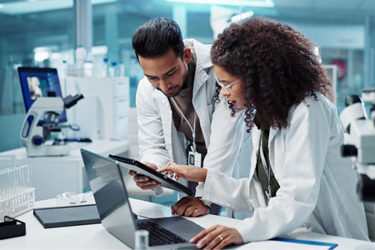
In the biopharmaceutical industry, there is a growing interest in improving the understanding of growth kinetics in mammalian cell-based processes, especially for monoclonal antibody (mAb) production. By employing mechanistic models or a first principle, it is possible to describe the complex mathematical and fundamental relationships within the cell as well as between the cell and its environment.
Although mechanistic models offer deeper insights into the process and help identify critical parameters, virtual representations of the process through digital twins can simulate experiments in silico. This approach reduces the workload and time required for process development and supports decision-making.
The focus of this study is to build a mechanistic model to determine the growth kinetics of a CHO-based process producing a mAb. After fitting the model with calibration data, it was tested on an independent validation dataset. Concurrently, an artificial neural network approach was utilized to determine specific productivity. These two modeling approaches were combined to create a hybrid model for predicting overall product concentration, forming the foundation for a digital twin.
Get unlimited access to:
Enter your credentials below to log in. Not yet a member of Drug Discovery Online? Subscribe today.