3 Ways Tissue Image Analysis Will Shape The Future Of Cancer Treatment With Immunotherapies
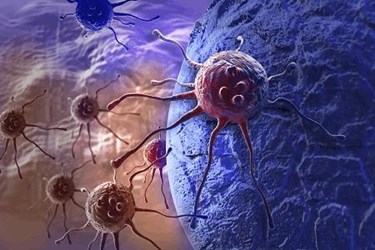
By Matthew Houliston, Definiens Inc.
As healthcare continues its transition towards precision medicine, physicians are eager to maximize both the quantity and quality of data obtained from their patients’ tissue samples. In the current “one drug, one test” paradigm, physicians struggle to balance the number of tests required to make a treatment decision with the limited amount of tissue that is available. Further complicating treatment decisions is the use of imperfect biomarkers associated with targeted therapies, as the patients who are identified to be ideal candidates for a targeted therapy respond only some of the time while patients who are not expected to respond do. This dilemma is of great concern for those who are responsible for paying for these expensive therapies - payers and patients – who would like to avoid unnecessary expenses for drugs that may not work and possibly cause debilitating side effects. Of course, of even greater concern is helping the patient identify the best therapeutic option that allows them the best opportunity to treat their disease and maximize their quality and quantity of life.
The use of image analysis holds the very promising potential to solve many of the issues surrounding patient selection for immuno-oncology (IO) treatments, particularly as more mono and combo therapies become available. With the ability to combine cognitive learning technology with sophisticated analytics assessing tumor and immune cells in context, image analysis of tumor tissue can identify complex signatures that incorporate intra- and intercellular features to better predict patient response for a given therapy or class of therapies. Image analysis will play a role for all stakeholders along the patient care continuum, including pathologists, physicians, pharmaceutical manufacturers, payers, and, most importantly, patients.
Automated, quantitative, objective image analysis to augment pathologists’ abilities
Image analysis promises to make the work of pathologists more efficient while augmenting their knowledge and expertise. Today’s companion and complementary diagnostics for IO therapies require the relatively simple exercise of manually estimating the proportion of cells that are positive for a given biomarker. If the proportion is above a certain threshold, the patient is considered a candidate for the associated drug. Yet, this is becoming a complicated road for pathologists to navigate. Currently, multiple drugs are associated with the same biomarker using different assays, different scoring criteria, different positivity thresholds, and even staining different cells (i.e., tumor vs. immune cells). The future promises more drugs, more drug combinations, and more tests. Unfortunately, pathologists’ ability to keep the drugs, tests, and testing specifics straight will only decrease as complexity rises, and this is before tests incorporate nuanced cellular features into intricate biomarker signatures.
Image analysis offers an automated solution using quantitative measurements of cellular features to objectively and accurately assess a patient’s tumor sample. Additionally, the capacity of the pathology lab to handle the increased volumes of tests as more patients are considered for treatment with novel IO therapies increases with the use of image analysis. This will be as a result of the automation of cell counting, algorithm application, and streamlined process utilizing a user-friendly interface that requires less thinking of the pathologists, thereby reducing complexity and opportunity for error.
Maximizing information obtained per sample
As single biomarkers prove to be insufficient for accurately predicting responders and non-responders of IO therapies, more sophisticated and complex biomarker signatures will be developed to extract greater amounts of relevant data from a sample. Even using today’s best technology supporting the manual nature of tumor analysis will be inadequate to accommodate the future use of complex biomarker signatures. Only through the support of image analysis will pathologists be able to reliably score tumor samples using complex biomarker signatures.
Furthermore, efficiently maximizing tissue information will continue to become more important as more tests are ordered for more treatment options, but the amount of available tissue sample stays the same. In order to obtain all of the relevant data to effectively use a complex biomarker signature, the use of sophisticated image analysis solutions will be required to discern differences between different stains on a single slide, co-register serial sections, identify necessary cellular features, and calculate spatial relationships and densities. Multiplexing is the surest way to accomplish this difficult feat, but only through the use of image analysis will pathologists be able to draw the most relevant conclusions to inform patient care.
Improving access to expensive IO drugs for the right patients
The high costs of IO therapies have put payers in a difficult position: they want to make these highly-effective drugs available for their population, but the finances do not permit them to do so. Pharmaceutical manufacturers who launch with a companion diagnostic strategy are more likely to see their therapies made available to patients, but given the high costs and the fact that these drugs may be used long-term, a patient selection strategy is not a guarantee of coverage (see UK NICE draft guidance for 2nd line use of pembro in NSCLC).
In addition to the cost and chronic use of these treatments, another important issue limiting access to treatment is that not all patients who are identified as likely responders to IO therapies are using today’s tests due to their unimpressive predictive values. Complex biomarker signatures identified using image analysis that have demonstrated high positive and negative predictive values will instill confidence in payers that the right patients are receiving the drugs. Those who the test identifies as not likely responding to a particular treatment will be put on another treatment to which they are more likely to respond, thereby offering the best chance at survival while limiting the waste of precious time and money. The health economics are substantially improved, and payers will facilitate access to even the most expensive therapies for the right patients.
Since the dawn of medicine, physicians have merged art and science to guide the diagnosis and treatment of disease. As more is understood about disease and more ways of diagnosing and treating disease become available, it is (and will continue to be) increasingly difficult for care givers to accurately navigate and apply the standard of care for each patient. As we continue to realize that disease is different between patients (e.g., what is causing the disease, how the disease presents, and how the body is responding), using data analytics will become more and more valuable. This is significantly evident in the fields of oncology and pathology, and applying the results of research using data analytics through the adoption of image analysis in clinical practice will ensure that the right patients have access to the best therapies for their unique disease.