Using AI In Drug Discovery Isn't The Golden Solution You Think It Is — Yet.
By Neil Dhawan and Jason Pontin, Totus Medicines
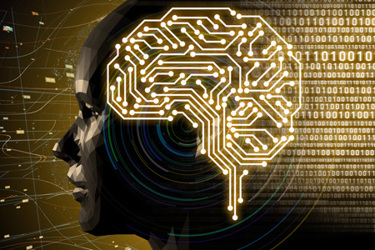
Despite the massive scope and investment of the pharmaceutical industry, and tremendous breakthroughs of modern medicine, 97% of the human genome and the proteins it creates cannot be targeted with existing drugs. Because 90% of the drug targets known to be driving human disease remain undrugged, most diseases, unsurprisingly, are left untreated.
Machine learning and artificial intelligence are heralded by the pharmaceutical industry and its investors as the keys to unlocking a better future for medicine with potential treatments for many untreatable diseases. But despite the impact of these technologies in other industries, current approaches to using AI in drug discovery miscalculate the central challenge. The key to using AI for drug development does not lie in building the right algorithm or amassing more computational power, but instead in obtaining enormous amounts of high-quality biological data that can be analyzed by somewhat conventional approaches.
The problem is that with current technologies, amassing high-quality biological data is prohibitively expensive. It’s possible to generate massive amounts of data, but the data tends to be low-quality and messy, leading to a well-known “garbage-in, garbage-out” challenge in AI/ML. AI/ML is only as good as the data it is trained on.
Before we realize the true potential of advanced AI and ML technologies to treat disease, we need to start by developing technologies that can generate the massive amounts of reliable, high-quality biological data that make up the fundamentals of any ML approach.
AI/ML Without Quality Data Just Creates Smoke And Mirrors
In 2019, researchers developed an AI model to design new drugs, and touted the discovery of new molecules for a well-known drug target called DDR1 in 21 days. The reality is that DDR1 kinase inhibitors were discovered 15 years ago, and many criticized this paper for overblown claims on a molecule that a graduate-level chemist could design in days,[1] not the weeks that the paper celebrates[2].
Similarly, in 2019, researchers at MIT developed an AI model that could predict the toxicity of drugs by analyzing their chemical structures. However, they found that the model was performing poorly, with high rates of false positives. Upon further investigation, the researchers discovered that the AI model had been trained on a data set that contained both toxic and non-toxic compounds, but the non-toxic compounds were structurally similar to the toxic ones. This led the AI model to learn patterns in the data that were not representative of the real-world toxicity of drugs and resulted in inaccurate predictions.
These studies highlight the “garbage in, garbage out” principle. Sure, these researchers developed a powerful algorithm, but without the right data, AI/ML could only rediscover findings that are already obvious at best.
Real-World Predictions Require Real-World Data, And A Lot Of It
In order to fully realize the potential of AI in drug discovery, we need to generate more high-quality data that accurately reflects the complex biological and chemical systems involved in drug development. Without a sufficient quantity and quality of data, AI models may be inaccurate, incomplete, or biased and may not provide the level of insight needed to accelerate drug discovery.
We need to build new technology that can measure how our body works at a molecular level across billions to trillions of data points. Imagine a search engine that can search and evaluate all the molecular interactions occurring in a cell when a patient is treated with a drug.
This does not require new code, algorithms, or computing power alone; it requires hard innovation in the real world, more akin to the invention of the printing press, electric cars, or airplanes.
We are finally starting to see real breakthroughs in biotechnology that could unlock the power of AI. Illumina, a biotechnology company, revolutionized genetic sequencing by developing next-generation sequencing (NGS) technology and has turned the cost of sequencing a genome from $10,000 about a decade ago to $200[3]. Illumina's NGS technology has transformed genomics research, enabling scientists to sequence entire genomes at unprecedented speed, accuracy, and scale. It has accelerated research in areas such as personalized medicine, cancer genomics, and infectious diseases. By enabling faster, cheaper, and more comprehensive sequencing, NGS has led to the discovery of new genetic markers, the identification of disease-causing mutations, and the development of targeted therapies.
Unfortunately, while genetic sequencing does help us understand our underlying biology, we need actual drugs to intervene in these diseases. Knowing why a disease occurs is not enough. We need a technology like next-generation sequencing for drug discovery that can search billions of potential drugs in cells to find the perfect molecule to drug the 90% of undrugged drug targets driving disease. Currently, our tools max out at screening only thousands of molecules in cells per day, too small to realize the potential of AI in drug discovery.
Companies like Moderna and AbCellera have embraced this challenge and have built core hard tech innovations to screen millions to billions of large molecule drugs. Similar innovations are needed in the oral small molecule drug space, which represent 90% of pharmaceuticals.
In conclusion, while AI has the potential to revolutionize drug discovery, its impact will be limited until we are able to generate more high-quality data that accurately reflects the complex biological and chemical systems involved in drug development. Without access to high-quality data, AI models may be inaccurate, incomplete, or biased, and may not provide the level of insight needed to accelerate drug discovery.
Technology that can solve this key problem and generate high-quality massive drug discovery data would help fuel a new era of AI-enabled drug discovery and disease treatment.
References
- https://www.science.org/content/blog-post/has-ai-discovered-drug-now-guess
- https://pubmed.ncbi.nlm.nih.gov/31477924/
- https://www.wsj.com/articles/illumina-launches-more-powerful-gene-sequencer-11664466535
About The Authors:
Neil Dhawan is co-founder and CEO of Totus Medicines, the first drug discovery company using chemical biology and AI to make the total human genome druggable. Dhawan’s mission for Totus and his team is to translate revolutions in chemistry, biology, and computation into covalent medicines at scale.
Jason Pontin is a partner at DCVC, where his belief that emerging technologies and new scientific insights can solve challenges and expand human possibilities inspires his investments in deep tech and life sciences companies. He was the first investor in DCVC-backed Totus Medicines, where he is a founding board member. Pontin also served as CEO and editor-in-chief of MIT Technology Review and editor of Red Herring magazine, and has written for a range of additional publications.