The Global Market For AI In Drug Discovery To Sextuple By 2027
By Anu Dhiman, MarketsAndMarkets
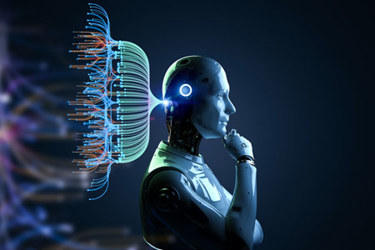
The integration of AI in drug discovery has been a game-changer, significantly improving efficiency and accuracy, and its use is growing exponentially. According to new analysis by MarketsandMarkets,1 the global market size of the use of AI in drug discovery was valued at $0.6 billion in 2022 and is expected to reach $4 billion by 2027, with a CAGR of 45.7% during the forecast period. Of the three major segments of the market (natural language processing, context-aware computing, and deep learning), deep learning accounted for the largest share in 2022. North America, as the early adopter, has captured the largest share of this market.
Major players operating in this industry include IBM Watson Health, Atomwise Inc., Insilico Medicine Inc., Exscientia Ltd., BenevolentAI Ltd., twoXAR Incorporated, among others that are investing heavily in developing innovative AI-based solutions that enhance drug discovery processes. Real-world examples of successful use of AI in drug discovery from these companies abound. For instance:
- Insilico Medicine used an AI-powered platform to identify a potential new treatment for fibrosis – a disease characterized by tissue scarring that has no cure currently.
- Atomwise employed deep learning algorithms to discover two drugs that could be effective against Ebola.
- BenevolentAI used AI to identify a potential treatment for idiopathic pulmonary fibrosis (IPF). After analyzing vast amounts of data and identifying key genetic targets, they were able to develop a new drug candidate called baricitinib. The drug underwent successful clinical trials and was approved by the FDA in 2018.
Let’s explore the market drivers, market constraints, and emerging trends of AI-powered drug discovery.
Market Drivers
The growth of AI in drug discovery has been driven by several factors. One of the key drivers is the need for more efficient and cost-effective drug development processes. With traditional methods taking years and costing millions, AI can significantly reduce both the time and cost involved. In particular, there has been increasing demand for new drugs and therapies due to the rising prevalence of chronic diseases such as cancer, neurological disorders, and cardiovascular diseases globally. By analyzing massive amounts of data and identifying patterns that humans might miss, AI can help researchers find promising drug candidates faster and more efficiently than ever before.
Another driver is the increasing availability of large data sets that are necessary for successful AI applications in drug discovery. The rise of Big Data has made it possible to collect and analyze vast amounts of information from a variety of sources, including electronic medical records, genomic databases, and clinical trial data.
Advancements in technology have also played a significant role as growth drivers. Machine learning algorithms have become increasingly sophisticated over time, making it possible to identify patterns in complex biological systems with greater accuracy than ever before.
Collaborations between academia, industry, and government agencies have helped drive growth by facilitating the exchange of knowledge and resources needed to develop effective AI tools for drug discovery. These partnerships have also helped speed up regulatory approval processes by providing valuable insights into potential safety concerns early in development.
Furthermore, favorable government initiatives to promote healthcare R&D activities, along with increased adoption of artificial intelligence technologies across various end use sectors worldwide, are also driving significant growth opportunities for this sector.
These growth drivers are expected to continue fueling expansion within this field as new technologies emerge and larger data sets become available for analysis.
Market Restraints
While the potential for AI in drug discovery is vast, there are also certain constraints on its widespread adoption. One of the primary challenges is the need for significant amounts of high-quality data with which AI algorithms can learn and improve. Without access to such data, machine learning algorithms cannot effectively identify patterns or predict outcomes.
Another challenge is that while AI can speed up certain aspects of drug discovery, it cannot replace traditional laboratory testing entirely. The development and testing of new drugs still require physical experiments on living organisms, a process that takes time and resources.
In addition, regulatory bodies like the FDA have yet to fully embrace AI as a means of drug discovery. There are concerns around transparency and accountability regarding how decisions made by machine learning models are arrived at – especially when compared with more traditional human-led methods.
There's also concern about a lack of diversity within data sets used by many companies in developing their algorithms - skewing results toward particular demographics - so further work must be done here, too, before widespread use becomes possible.
These constraints highlight both the promise and limitations of using AI in drug discovery today, but as technology continues to evolve over time we will continue seeing progress being made toward realizing this future vision.
Emerging Trends And Technologies In AI And Drug Discovery
AI algorithms are being developed to analyze complex biological systems across multiple scales, from molecular interactions to tissue-level responses. These advances allow for more accurate predictions of how drugs will interact with human cells and tissues. Through machine learning algorithms, scientists can simulate how drugs might affect different patients based on their genetic makeup or other factors. This predictive power could help researchers identify possible side effects early in the development process, potentially saving time and money by avoiding late-stage failures due to unexpected toxicity issues.
Generative models are being used to design entirely new drugs. These have a knowledge structure of the molecules that are known to have therapeutic effects, allowing them to generate candidates that meet specific criteria. These candidates can then be screened for their efficacy and safety, leading to the discovery of new drug classes.
Cloud computing is another technology that supports drug discovery. By enabling computation at scale, cloud-based infrastructures have significantly reduced the time and cost associated with drug discovery. Researchers can access resources without building their own computing infrastructure, allowing them to focus on their primary task of drug discovery.
Virtual drug screening is making substantial contributions to drug discovery. By simulating the interaction between potential drug candidates and disease targets, researchers can screen thousands of compounds quickly, speeding up the drug discovery process.
Lastly, integrative approaches have revolutionized the drug discovery process. By bringing together diverse sources of data, such as molecular, genetic, and clinical data, researchers can gain a comprehensive understanding of the disease biology and identify potential drug targets. This approach reduces the risk of later-stage clinical failures, resulting in the discovery of more effective and safe drugs.
Final Thoughts
The future looks bright when it comes artificial intelligence enabled drug discovery; however, it is important that businesses take steps now to ensure they are prepared to capitalize on this technology’s full potential when it comes time to implement these solutions in their operations. This includes investing in research & development as well training personnel on how best to utilize these technologies.
Reference
About The Author:
Anu Dhiman is associate director, healthcare, at MarketsAndMarkets. She has more than 16 years of experience in offering development, strategic consulting, and advisory services. With a focus on the healthcare and life science industries, her expertise spans across pharmaceuticals, biotechnology, medical devices, healthcare IT, digital health, and health services. Dhiman has a bachelor’s degree in pharmacy and holds an AGMP certification from IIM Ahmedabad, India.