Tapping Into AI/ML Partnerships To Advance Drug Discovery With Recursion Pharma
A conversation with Recursion Pharma CEO Chris Gibson
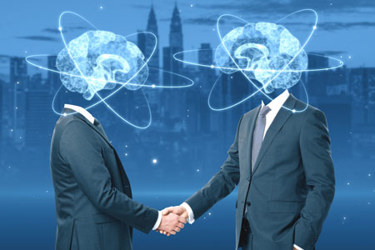
Ask just about anyone in clinical research — from those working in data management to clinical operations to regulatory affairs — about trends they’ve been following, and almost all will mention the progression of artificial intelligence (AI) and machine learning (ML) in advancing drug discovery and development.
And how could they not? AI/ML has pervaded all facets of our lives; we can’t seem to escape all the ways in which folks play with ChatGPT and other AI chatbots. But perhaps one of the areas of most critical interest is the drug development space. The potential of AI/ML has certainly not been lost on Recursion Pharma, which launched in 2013 and recently scooped up two Canadian tech companies earlier this month: Valence and Cyclica.
Headquartered in Salt Lake City, with offices in the Bay Area and Canada, Recursion is a clinical stage “TechBio” company industrializing drug discovery by leveraging AI/ML. Recursion has entered agreements to acquire Cyclica for $40 million and Valence for $47.5 million.
To learn about the acquisitions and the future of AI/ML in drug discovery and development, we caught up with Recursion’s CEO Chris Gibson.
Recursion calls itself a “TechBio” company. While we often come across “biotech” in the clinical research space, the inverse of the phrase isn’t as prevalent. What’s the story behind choosing that descriptor?
GIBSON: TechBio is a term that emerged a few years ago and is quickly gaining popularity. It’s intended to create a distinct category for the growing number of companies in our industry that have an explicit strategy of using technologies like computation (and particularly machine learning and artificial intelligence) and automation to do drug discovery differently.
In the past year, we at Recursion have noticed a shift in the continued acceleration of people’s appreciation of the potential for the TechBio space. From large pharmaceutical companies to large technology companies, it feels to us like there is a growing sense of inevitability among leaders that these companies that have technology at their core will indeed create step-function shifts in the healthcare industry — an opinion that has not been widely accepted until recently.
Given your moniker, it’s no surprise that Recursion decided to bring more AI/ML capabilities in-house. Just this month, Recursion announced its decision to acquire both Cyclica and Valence. What capabilities do they bring?
GIBSON: With the agreement to acquire these two companies — both of which are developing machine learning methods and models to transform drug discovery — Recursion will significantly bolster our chemistry and generative AI capabilities. Valence develops generative AI and deep learning models for the application of low-data learning in drug design. Cyclica also occupies the AI-enabled drug discovery space and has pioneered a deep learning engine that predicts the polypharmacology of small molecules through prediction of ligand-protein interaction as the foundation for small molecule drug discovery.
Our focus for the last decade has been on using technology tools to map and navigate biology as a system, whereas these companies have had a chemistry-first approach. Through these acquisitions, we intend to create the first full-stack technology solution capable of industrializing the drug discovery process, end-to-end: from in-house data generation to identifying novel biological targets to designing and optimizing chemical compounds — all at massive scale.
All the while, AI/ML is increasingly the subject of interest and conversation in clinical research and drug discovery. To start, what do we know about each’s potential for advancing drug discovery? And what can it enable that can’t be done — or can’t be done well — without it?
GIBSON: There are two primary ways AI and ML can impact drug discovery. The first is increasing efficiency — think faster, cheaper, and less failure. Several companies — Recursion included — have already demonstrated that’s possible. The second is revealing something completely new about biological function that we as humans can’t do on our own. Combine both efficiency and novelty – that’s the true opportunity for us to industrialize drug discovery.
One of the biggest lessons learned from other industries where these technologies have already made a significant impact is that developing an amazing machine learning algorithm alone is rarely sufficient to create disruptive change. Outsized change is created when these sophisticated algorithms are paired with the right data, typically in an iterative process of ongoing learning, prediction, and refinement. At Recursion, we’ve generated our own massive proprietary data set in-house, fit for the purpose of ML, rather than repurposing existing internal or public data sets.
Recursion and Valence’s Scientific Advisor Yoshua Bengio has said that these acquisitions will bring about some of the “most compelling AI/ML research in the world.” That’s a bold proclamation. How will bringing all three companies under one roof achieve just that?
GIBSON: Yoshua is a visionary and a Turing award winner, and so it is exciting to us that he shares our excitement about what is possible here at the intersection of biology and technology. First, Recursion has built one of the largest biological data sets on Earth at 23 petabytes. That’s more data than every movie ever made put together, multiple times over. This data is essential as a substrate on which to build machine learning models that can help us understand biology and chemistry.
Another key ingredient is compute power, and Recursion operates one the 150 fastest supercomputers on Earth, so we can check that box.
And the third most important ingredient is people. We are always on the lookout for exemplary AI/ML talent to bolster our team, and these acquisitions will bring top talent to Recursion. Yoshua and the team at Valence are based at Mila, one of the largest and most innovative deep learning research institutes worldwide, and the team at Cyclica has long been at the forefront of deep learning research for small molecule drug discovery.
Understanding that AI/ML has the potential to revolutionize the drug discovery process, what are the implications for success in conducting clinical trials? Can we expect more trials that began with AI/ML-led drug discovery to perform differently — in terms of safety and efficacy — than those that do not?
GIBSON: Biology is incredibly complex, and most programs fail in the clinic because we don’t pick the right target. Since our founding in 2013, Recursion has focused on solving this problem by leveraging the large-scale interrogation of systems-level biology and building one of the world’s largest biological and chemical data sets. This has enabled us to predict trillions of relationships across biology and chemistry in our Maps of Biology, leading us to identify novel biological targets and chemical starting points that may help us find new ways to address difficult-to-treat diseases.
My hope is that in the coming years, as our industry continues to accelerate and improve these technologies, we will start to reduce the failure rate of clinical programs because we will get better and better at selecting the right targets and the best molecules to modulate those targets early in the process. There are also many early forays of machine learning into trial design, patient selection, and more. Najat Khan, chief digital officer of Janssen, has deployed tools there in clinical development and commercialization to great success.
All clinical trials intend to determine a therapy’s safety and efficacy. For Recursion, the part of that goal is to also be transparent about those findings. The company’s policy is to make all trial results public within a year of the trial’s completion date and to share results with patients upon request. Why is it important to Recursion to exhibit transparency in communicating its results, good or bad?
GIBSON: I feel that candor is a critical ingredient in achieving our mission. We’re in this for the long haul, so creating a track record of trust and transparency with the folks who are on this journey with us is essential. Recursion also has a strong commitment to open science and releasing open-source data sets, which we believe drives value for us and society as a whole. To date, we’ve released five open-source data sets, some of which are the largest of their kind in the world, under terms that allow for broad academic and commercial use. Our goal is to enable the next generation of machine learning methodologies to foster research, methods development, and collaboration.
What’s next for Recursion? Is it getting settled with the two additions, setting sights on another acquisition, or something else altogether?
GIBSON: We’re proud of what we’ve achieved, especially in an environment where we’re seeing a lot of consolidation and rapid change in the industry. Our acquisitions of Cyclica and Valence add capabilities in digital chemistry, as well as machine learning and artificial intelligence. Combining their skillsets with our large-scale automated wet laboratories and supercomputing capabilities, we’re able to deploy what I believe is the most complete, technology-enabled drug discovery solution in the biopharma industry.
Looking ahead, we’re focused on integrating these capabilities into our existing drug discovery engine, which I believe we can do very quickly given how complementary these technologies are to what we’ve built at Recursion. We will deploy this full-stack approach against our existing pipeline to rapidly accelerate programs into the clinic.
About The Expert:
Chris Gibson, Ph.D., is the cofounder and CEO of Recursion, the clinical stage TechBio company decoding biology to industrialize drug discovery. Chris developed the technology and approach that seeded Recursion as part of his MD/Ph.D. work in the lab of cofounder Dr. Dean Li (now president of Merck Research Labs) while at the University of Utah. After completing his Ph.D., Chris left medical school to launch Recursion. Chris is a graduate of Rice University with degrees in bioengineering and management. Chris serves on the board of BioUtah and is the chair of BioHive, the public-private partnership driving expansion of Utah's life sciences ecosystem. Chris is also active as an advisor and mentor, both formally and informally, of many young biotech founders.