3 Recommendations For Improved AI/ML Models In Drug Discovery
By Younes Amar, AI/ML product leader, Wallaroo Labs
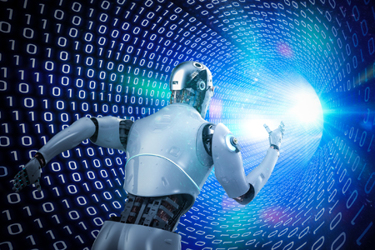
Drug discovery is a long, expensive, and risky process. Although the last few years have given us incredible examples of the speed at which vaccines and antivirals can be delivered to market, data from industry group PhRMA suggests that on average it takes 10–15 years and costs $2.6 billion to develop one new medicine. This includes the cost of many failures along the way.
However, despite intensive time and resource requirements, the pharmaceutical drug discovery technology market is expected to grow from $53.3 billion in 2021 to $80.2 billion by 2026. Much of this growth is driven by the potential profit of new drugs. AHIP notes that pharmaceutical manufacturers earned an average of $18.6 billion in total global revenue for new drugs, creating tremendous incentive for manufacturers to find ways to reduce costs and limit the failures that often accompany the traditional trial and error discovery process. These incentives, and the process of drug discovery trial and error, have led many drug manufacturers to explore and implement emerging technologies such as artificial intelligence (AI) and machine learning (ML) modeling in the drug discovery process.
A recent report from The Business Research Company projects 30 percent growth in AI adoption for drug discovery. This makes sense when you consider how AI and ML approaches can offer more targeted insights and decision-making for well-specified scientific or medical questions involving abundant large complex data sets. ML models can be applied throughout the drug discovery process, including target validation, identification of biomarkers, and designing more effective clinical trials.
However, AI/ML initiatives are not without their own challenges. For example, AI/ML models in life sciences tend to be complex as they run on data sets that comprise clinical, genetic, and genomic data. Additionally, they require high levels of interpretability and repeatability as they transition from the early exploratory/feasibility stage to the clinical trial and manufacturing stages. Keeping this in mind, drug manufacturers need to make sure their AI-based approach is 1) actionable, 2) transparent, and 3) repeatable in order to optimize their R&D and commercialization efforts.
1. Actionable AI
Drug discovery involves a tremendous number of variables. These can include chemical makeup, drug properties, and molecular interactions, just to name a few. When combined, these variables can create millions of results and metrics that need to be analyzed throughout the process. As a result, nearly 90% of clinical trials fail in the first phase as researchers lack the efficiency and tooling to identify and address the root causes behind those failures.
For example, there are estimated to be in the range of 1030–1060 drug-like molecules, meaning millions of combinations of attributes would need to be tested to achieve an effective treatment with minimum side effects or adverse events. Producing such inferences takes a tremendous amount of time; time that the industry doesn’t have.
Fast and efficient data analysis leads to effective decision-making on whether a specific drug candidate is worth pursuing. That can potentially save companies millions (or billions) of dollars in wasted R&D and shorten timelines for bringing new drugs to market.
2. Transparent AI
Additionally, meaningful actions and governance on AI-enabled decisions can be achieved with effective observability and monitoring (including proper management of anomalies and bias with full ability to explain). As of 2019, the FDA has been adopting a modified regulatory framework for AI/ML-based software products as medical devices. As a result, manufacturers are expected to provide transparency and real-world monitoring of their AI-enabled algorithms or software as a medical device. By extension, this means that drug manufacturers need the ability to monitor and explain AI algorithms from pre-market development (including during drug discovery) to post-market performance.
The most successful ML models are designed with a framework to detect model or concept drift by benchmarking distributions to simplify the identification of target attributes (calculating a baseline distribution and measuring how future distributions fall within these results). Advanced ML observability can quickly identify abnormalities, allowing researchers to troubleshoot models and effectively identify root causes behind anomalies and biases. This creates a transparent feedback loop that allows researchers to tune their scoring mechanisms and focus on other areas that may have previously been overlooked.
3. Repeatable AI
One of the major challenges in the drug discovery process is the sheer scale and complexity of data sets involved in the drug development process. This scale makes it difficult to achieve repeatability in processes that can continuously produce and operationalize data insights in an effort to optimize R&D efforts.
The current drug discovery process is error prone without enough data to validate it. Additionally, the emergence of genomics and the impact of RNA technology on scientific research have increased the variables that scientists can explore for potential drugs and their efficacy. ML frameworks can be designed to allow scientists and researchers to iterate effectively on their research and discovery initiatives. ML frameworks running on large-scale data sets enable researchers to achieve more tangible answers to their scientific questions or hypotheses. This, in turn, helps models used in drug development to learn and make sense of these complex behaviors such as mining heterogeneous causal effects to develop personalized cancer treatments as can be seen in the figure below.
Workflow of the application of Survival Causal Tree. Reused with permission from Zhang et al., 2017.
Improving ML In Drug Discovery
AI/ML utilizes systems and software that can interpret and learn from the input data to make independent decisions for accomplishing specific objectives. These models offer drug manufacturers greater potential for rapid decision-making, reduced costs, and improved time-to-delivery.
As technology improves and processing power increases, AI/ML will further level the playing field in drug discovery; however, due to the very real life and death consequences associated with new drug application, these models must be precise, easily scalable, and able to adapt as new information and data sets become available. By optimizing the speed of deployment and accuracy of the ML models finding their way into the R&D process, drug manufacturers can unlock the true potential of AI/ML.
About The Author:
Younes Amar is the head of product at Wallaroo Labs. Prior to joining Wallaroo, he was the data science and AI product lead at Tempus Labs. Amar’s experience is in software engineering, product strategy, and development with focus on analytics and ML platforms in various industries such as ESG, government, logistics, healthcare, and insurance.