Opportunities For AI To Assist Cell & Gene Therapy Companies
By Yoshio Hagiwara, Paolo Siciliano, and Willem Van Asperen, PA Consulting
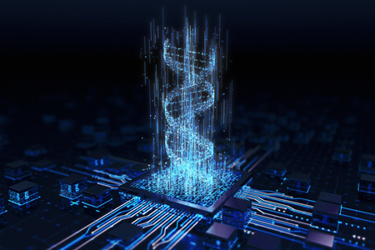
The cell and gene therapy (CGT) sector saw remarkable progress in the last few years, with a dramatic increase in the number of pipelines and approved products. However, despite the considerable advances, the CGT market is still facing numerous challenges that are hindering its growth and delaying the broad uptake of these therapies in the healthcare system. Examples of challenges in this space include the limited understanding of certain diseases, the complex nature of these therapies, and limitations in the scale-up and optimization of manufacturing processes. Driven by advancements in digital technologies and computing power as well as the proliferation of data, we are witnessing artificial intelligence (AI) and machine learning (ML) solutions being applied to the CGT sector. The hope is to overcome the challenges preventing mainstream adoption by analyzing and deriving insights from large amount of data. Although AI/ML is still in a nascent stage in the CGT sector, we expect the technology to transform both the ecosystem and value chain of CGT.
AI’s Potential Impact On The Pharma R&D Business Model & Investment Strategy
AI is expected to revolutionize the CGT sector in a way similar to how it disrupted the traditional pharma industry for small molecule development and manufacturing.
The CGT ecosystem consists of a complex network of interactions between a number of stakeholder types, including major pharma, biotech companies, equipment manufacturers, software developers, and healthcare providers. In the last few years, several AI and ML technology vendors have emerged to collaborate with CGT developers and manufacturers to address challenges through advanced data analytics and AI-based tools. This is unleashing new opportunities in the area throughout the CGT value chain from early R&D to post-commercialization.
One of the areas where AI is expected to make a great impact is in the transformation of the pharma R&D business model. The model of costly and years-long end-to-end development of blockbuster drugs by large pharma is gone. Now, major innovation in drug discovery and early R&D are driven by small companies, while major pharma provides the investment and resources for clinical development and commercialization of new therapies. This trend also can be observed in the CGT sector, where some large pharma companies are selling their early-stage R&D programs (for example, Pfizer is planning to sell its preclinical rare disease gene therapy portfolio this year), while partnering with or acquiring smaller companies that have succeeded in bringing their pipeline products to the clinical stage. This symbiotic relationship is likely to continue, providing benefits to both parties. As highlighted in this article, AI and ML are poised to accelerate early R&D, while minimizing the associated cost and risk. Having access to such technologies will be key for small CGT developers to attract investment from highly risk-averse major pharma. For this reason, we expect to see an increasing number of partnerships between small CGT developers with AI capability and large pharma, resulting in faster development and commercialization of new therapies.
Regulators are another key stakeholder in the CGT ecosystem to react to the rapid advance in AI technologies. Regulatory bodies of major countries started to lay out guidelines and frameworks to safeguard and prevent negative impact of AI (e.g., protecting data privacy, preventing AI bias), which may offset innovation in this space. The EU Commission, for example, has released a proposed AI Act aimed at improving patient health and eliminating possible algorithm bias, stating that the data sets used to develop AI must be representative of the intended patient population. In the U.S., the FDA has released the Cybersecurity Modernization Action Plan, with a goal of “strengthening the FDA’s ability to protect sensitive information, modernize cybersecurity capabilities, and improve situational awareness to decrease overall security risks to the Agency.” Currently, there are ongoing debates on how to achieve a fine balance between AI innovation and AI-associated risks, and thus we expect to see continuous evolution in AI-related laws and regulations to accommodate both.
AI solutions can find applications across the whole CGT value chain, from early R&D to post-commercialization. Below, we explore how AI and ML solutions can accelerate innovation in CGT while addressing challenges in different stages of the value chain, showcasing some interesting examples found in the industry.
Acceleration Of Early R&D
AI and ML can facilitate the discovery of a drug target by efficiently handling high-throughput, heterogeneous, and complex molecular data, extracting the feature or relationships in biological networks. For example, AstraZeneca identified a novel target for idiopathic pulmonary fibrosis (IPF) through its collaboration with an AI drug discovery company, BenevolentAI. Using BenevolentAI’s natural language processing (NLP) tool, AstraZeneca has integrated publicly available disease-specific data as well as its proprietary data from a mixture of unstructured and structured data sets into the BenevolentAI Knowledge Graph.
Another area where AI and ML can provide additional support to CGT developers is in the optimization of the delivery methods for gene therapies. Today, most gene therapies are delivered via viral vectors. While they are an efficacious means of gene delivery, viral vectors are prone to be targeted by the host’s immune system, causing immune reactions in patients and, as a result, rendering the therapy less effective. It is reported that almost 50% of patients are currently excluded from gene therapy treatment due to preexisting immunity to the viral capsids.
In collaboration with Google Research, Harvard’s Wyss Institute for Biologically Inspired Engineering, and the Harvard Medical School laboratory, Dyno Therapeutics applied deep learning to develop an AI platform capable of designing adeno-associated virus 2 (AAV2) variants with optimum immunity-evasion property. Nearly 60% of the variants produced by the AI platform were determined to be viable, a significant increase compared to fewer than 1% when produced using the traditional mutagenesis method. In addition to immunity evasion, this AI platform is also claimed to be capable of designing viral capsids with optimum targeting ability, payload size, and manufacturability. Dyno Therapeutics is currently working with key gene therapy players such as Novartis, Sarepta Therapeutics, and Roche and its subsidiary Spark Therapeutics to help supply a new generation of more effective and flexible AAV vectors.
Acceleration And Facilitation Of Clinical Trials
Most CGT therapies tend to target rare diseases, for which patient recruitment can be extremely challenging. In addition, most of these disorders are generally life-threatening or life-limiting, and the use of placebo control arms in clinical trials is often considered unethical. AI can be used to identify suitable patient cohorts for clinical trials. For example, NLP can analyze unstructured data from medical records or patient health records to identify patients with specific symptoms, biomarkers, and/or diagnoses that match clinical trial inclusion criteria. In this case, the recruitment process can be accelerated by alerting healthcare professionals and patients about trial opportunities as well as by simplifying entry criteria in an accessible manner for patients.
In addition, an external control generated through statistical methods using external patient-level data, known as synthetic control arm (SCA), can be used to reduce the time, costs, and practical and ethical hurdles of employing control arms in clinical trials. SCAs are created primarily from observational real-world data (RWD) and previous clinical trial data. Although still in early stages, NLP and ML can be leveraged to analyze both structured and unstructured clinical data to create SCAs, providing CGT manufacturers with an opportunity to reduce cost and time of clinical trials in addition to overcoming such ethical challenges.
Optimization Of Manufacturing And Scale-up
As CGT products are generally sourced from living cells, they are prone to alteration and variation during the manufacturing process, and thus maintaining lot-to-lot consistency is a bigger challenge compared to the traditional small molecule-based pharmaceutical products. Examples of lot-to-lot variations in CGT include cell count, viral titres, and number of full vectors vs. empty vectors, among several others. Identifying the optimum manufacturing conditions to yield products of high quality with consistency requires extensive optimization processes and data analysis. Data-driven predicting models, such as digital twins, can simulate cellular and viral behavior in full-scale bioreactors based on different conditions, such as mixing patterns, gas exchange, and media compositions, which can then be quickly analyzed by AI models for real-time optimization.
Applying digital twins in the manufacture of products derived from living organisms has already been explored in the food industry. For example, the nutrition ingredient supplier Royal DSM has opened an AI lab in the Netherlands to optimize the culturing process of microorganisms such as algae, fungi, and bacteria for the production of nutritional molecules such as vitamins and lipids. This concept could potentially be transferred to CGT manufacturing processes to improve efficiency and consistency. Using Ansys’ (Pennsylvania, U.S.) simulation solutions and digital twins, Antleron (Leuven, Belgium) designs and develops custom bioreactors for cell growth and for real-time, non-invasive monitoring of the cell growth during the process itself.
AI and ML are also currently being developed and/or tested for a wide range of applications within CGT manufacturing, from cell media optimization (Cytiva) to increasing viral vector productivity (4x Bio), quality control (Coriolis Pharma), and more.
Optimization Of Operation And Supply Chain
CGT developers tend to rely on technologies and processes from early development work, which may not be suitable for efficient large-scale manufacturing. Implementing a robust, efficient, and reproducible platform for therapy manufacturing is essential to enable commercial scale. AI technologies such as generative AI can identify inefficiencies in manufacturing and optimize processes and workflow by analyzing a large amount of data related to process parameters, equipment settings, and product quality.
Even when a manufacturing process is fully optimized, the production of therapy products may halt due to constraints in the supply chain. CGT supply chains face a number of logistical issues such as quality and reliability of raw materials, volatile demand for CGT products (affected by parameters such as availability, cost, reimbursement policies, and change in patient treatment or patient condition), cold supply chain demands, and high costs. AI can be used to analyze data related to demand, inventory levels, and logistics as well as to optimize inventory levels according to demand, track product movements, and find opportunities to reduce transportation time and improve delivery time. In addition, AI solutions also can be applied to predict supply chain disruption through analysis of data related to shipping schedules, traffic loads, weather, etc., allowing manufacturers to plan contingency measures to minimize the disruption.
Supporting Regulatory Compliance
Managing and meeting regulatory compliance is typically manual work, requiring analysis and management of massive amounts of data and documents. Therefore, this process is error-prone and time-consuming, requiring substantial numbers of personnel. AI and machine learning are emerging as ground-breaking technologies to facilitate and semi-automate this process.
For example, AI can accelerate regulatory submissions and time to market for novel therapies. Through the analysis of large amounts of regulatory submissions, the AI can automatically generate regulatory documents from templates or extract data from clinical trials, allowing manual work to be focused on more complex tasks, thus accelerating regulatory submissions. Based on a vast amount of regulatory-related data stored in a company, AI can also predict queries that regulators may ask, thus allowing the company to prepare for these potential requests in advance, minimizing back-and-forth communication between the company and regulators.
In addition to regulatory compliance and submission, AI technologies such as NLP and large language models (LLMs) can semi-automate regulatory intelligence, which is labor-intensive and time-consuming, requiring regular checks of websites of regulatory agencies.
As shown above, AI has great potential to address challenges throughout the CGT value chain by enabling navigation and analysis of large and complex data, automating and speeding up manual and error-prone processes and workflow, and allowing trials of various options and conditions in virtual settings simultaneously. This transformative technology is already redefining industries worldwide, and it will eventually catalyze transformation in CGT sector. Especially for small CGT developers, having AI capability to reduce the cost and time frame of R&D will attract investments necessary to develop and bring therapies to patients. Stakeholders operating in the CGT space must consider the advantages and opportunities that AI may bring to their businesses and start developing strategies for implementation, since adoption of AI is not merely purchasing a tool from vendors but requires a comprehensive change in organizational structure and operation. While AI technologies are promising for R&D and post-commercialization stages, they still face significant challenges that need to be overcome. These will be discussed in more details in our subsequent article.
About The Authors:
Yoshio Hagiwara is a life sciences expert at PA Consulting. He has worked with and supported a number of pharma and medtech companies in technology innovation and development. His main areas of expertise include the application of data analytics and digital technologies in healthcare. Yoshio has a MSc in drug discovery and a Ph.D. in biochemistry.
Paolo Siciliano is an associate partner and life sciences expert at PA Consulting and leads PA Consulting’s work in CGT globally. He has several years of experience in supporting major pharma, biotech, and medtech companies to identify, develop, and leverage new technologies to solve business needs, as well as improve their innovation and product development processes. His main areas of expertise range from technology and commercial strategy to technology development, across a number of therapeutic areas. Paolo obtained a Ph.D. in molecular biology and worked as a senior research scientist in biotech companies in the U.K.
Willem van Asperen is the chief data scientist at PA Consulting. He has more than 20 years of experience in solving business challenges by applying a broad range of software engineering, data analytics, and artificial intelligence (AI) techniques. With a background in computer science and specializing in machine learning (ML), robotics, and neural networks, van Asperen develops predictive models for clients across a range of sectors, including healthcare and life science, public services, finance, and energy & utilities. He leads PA Consulting’s data science capability across multiple geographies and is an executive lecturer at Nyenrode Business Universiteit.