Navigating The Murky Waters Of Patent Claims Involving AI After Amgen v. Sanofi
By David McCombs, Dina Blikshteyn, Eugene Goryunov, and Matthew Beck
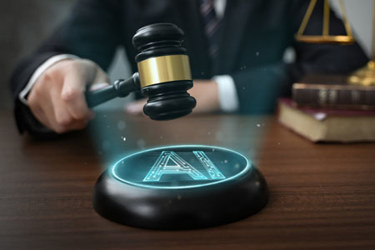
In May 2023, a unanimous U.S. Supreme Court in Amgen Inc. v. Sanofi1 issued a ruling on the Section 112 enablement requirement for patents. Section 112 requires a patent applicant to describe the claimed invention “in such full, clear, concise, and exact terms as to enable any [person of ordinary skill in the art2…to make and use the [invention].” While patents in Amgen pertained to antibodies, the Court’s decision is likely to have implications in other areas, including artificial intelligence (AI) systems.
Background
In 2014, Amgen obtained two patents that claimed an entire genera of antibodies that bind to specific amino acids and help reduce levels of cholesterol. Amgen identified the amino acid sequences of 26 antibodies that perform the claimed functions — what Amgen called its “roadmap” technique — and disclosed two methods of making other antibodies that also perform those functions — what Amgen called its “conservative substitution” technique. The “roadmap” technique directed scientists to generate and test a range of antibodies through several processes of elimination to determine whether they perform the functions described in the claims.3 The “conservative substitution” technique directed scientists to start with an antibody known to perform the claimed functions, replace the amino acids with amino acids known to have similar properties, and test the resulting antibody to see if it performs the claimed functions.4
Supreme Court’s Holding
The Court began with a review of the enablement requirement of Section 112. The enablement requirement mandates that a patent applicant describe the claimed invention “in such full, clear, concise, and exact terms as to enable any [person of ordinary skill in the art [POSITA]] … to make and use the [invention].” This requirement has existed since the Patent Act of 1790, and while the statutory text has changed over the years, the basic concept remained the same: “the specification must enable the full scope of the invention as defined by its claims. The more one claims, the more one must enable.”5
When it comes to claims covering an entire genera, or class, “the patent’s specification must enable a person skilled in the art to make and use the entire class.”6 The Court explained, however, that the enablement requirement cannot be applied rigidly. It does not require, for example, that the specification always “describe with particularity how to make and use every single embodiment within a claimed class.”7 Indeed, the sufficiency of “enablement is not measured against the cumulative time and effort it takes to make every embodiment within a claim.”8 Thus, a patent specification describing a general quality of a class may be sufficient to meet the enablement requirement where the specification reliably enables a person of ordinary skill in the art to make and use the entirety of what is claimed.9
In this case, the Court found that the specifications of Amgen’s patents did not provide techniques enabling the entire genera recited in the claims. Instead, the Court described the roadmap and conservative substitution techniques as “research assignments” for scientists to perform, each requiring extensive experimentation to see what works.10 The Court declined to develop a bright line rule for what constitutes sufficient enablement, but its decision leaves one to wonder what is sufficient disclosure to satisfy enablement in claims covering classes and what experimentation, if any, may be acceptable. On the one hand, a specification that requires some degree of experimentation may be acceptable, while on the other, a specification that requires a person of ordinary skill in the art to engage in trial and error may be insufficient.
The Reach Of Amgen For Patent Claims Involving AI
While Amgen specifically dealt with an antibody patent, the Court’s decision is likely to have implications across the patent landscape. For instance, patent claims involving AI may, similar to the claims in Amgen, face enablement and sufficiency of disclosure challenges.
AI inventions cover a broad array of software technologies. Early AI systems were written to imitate human reasoning through a set of preprogrammed instructions or scenarios, though many implementations today incorporate an adaptive, or learning, element. AI systems are “black boxes.” Training data sets are used to train the black boxes to identify patterns in the training data, sometimes with human supervision and interaction, and sometimes through rewards for providing desired outputs based on input training data. This leads to a number of questions.
Will such training data need to be disclosed in a patent application following Amgen? Since various AI systems may be utilized for many purposes, training data plays an important role in achieving desired results. Accordingly, it may be necessary under Amgen to disclose how training of the AI was performed, the training data sets used, and the different weights applied to the data within the data sets to enable a patent implementing an AI system.
Is disclosure of training data necessary and, if yes, would it be sufficient? Under Amgen, one could argue that providing training data would merely act as instructions to create an AI system that is capable of performing the claims, similar to Amgen’s self-described “roadmap.” The training data provides a means to achieve the AI system but may require training, testing, and experimentation to reach a similar system to what is claimed. It is therefore unclear whether disclosing the training data and data sets that trained the AI system would be enough. One may argue that details of how the training data was used and the underlying operations of the AI system must be disclosed.
Implications For AI’s “Black Box”
At a fundamental level, AI is a black box. Data goes in, and an output is generated. However, it is not necessary for an individual using or training an AI system to understand how the AI processes the data, determines the output, or the structure itself that is inside the black box.
An inventor may incorporate AI into their invention without understanding how the underlying AI actually works. In fact, in many instances a pretrained AI system is provided by a third party, and an inventor may not even know how the AI system works or was trained. When using an AI system, the underlying AI structure inside the black box does not need to be seen or known. Accordingly, one’s knowledge may be limited to the inputs and the outputs. This may present challenges under Amgen, as it may not be possible to provide extensive details in the patent specification to enable the claimed invention beyond data related to inputs and outputs or the AI system’s usability.
And while it may be desirable to disclose how outputs are generated by an AI system, such disclosure may be difficult, if not impossible, to provide. Outputs may have a certain meaning based on how the AI system was trained, and different training or use of different training data may result in different outputs. Moreover, outputs are based on probabilities or a range of probabilities. These probabilities differ for different data sets. Introducing information related to outputs, such as ranges determined by the AI or probabilities that correspond to the selected outputs, may have undesirable impacts on the scope of the claims or present further enablement issues.
While Amgen does not purport to upend enablement requirements, practitioners will want to be aware of potential implications for practice across every field, including AI. Until a definitive impact is determined, applicants will want to ensure that their patents include details that amount to more than research instructions to ensure that claims survive prosecution and are not struck down under Section 112 during litigation. And when drafting claim sets, applicants will want to be aware of the breadth of claim sets, as broader claims will face more scrutiny under Amgen than more narrow or targeted claims.
This article reflects only the present personal considerations, opinions, and/or views of the authors, which should not be attributed to any of the authors’ current or prior law firm(s) or former or present clients.
References
- No. 21-757 (May 18, 2023).
- A person of ordinary skill in the art is a hypothetical person who is presumed to have known the relevant art at a time a patent application was filed. See M.P.E.P. 2141.
- Id. at p. 5.
- Id. at p. 5-6.
- Id. at p. 13.
- Id. at p. 13.
- Id. at p. 13.
- Id. at 18.
- Id. at 13-14.
- Id. at 16.
About The Authors:
David McCombs is a partner in the Intellectual Property Practice Group at Haynes Boone. He is primary counsel for many leading corporations in inter partes review (IPR) and is regularly identified as one of the most active attorneys appearing before the Patent Trial and Appeal Board (PTAB). He has extensive experience advising clients with a wide range of IP matters, including appellate argument, patent litigation, and portfolio development. He can be reached at david.mccombs@haynesboone.com.
Dina Blikshteyn is a partner in the Intellectual Property Practice Group and a co-chair of the Artificial Intelligence Practice in the New York office of Haynes Boone. Her practice focuses on post-grant proceedings before the U.S. Patent and Trademark Office, preparing and prosecuting domestic and international patent applications, and handling trademark and other IP disciplines. Blikshteyn specializes in AI in technology and software areas and speaks regularly on the topic. She can be reached at dina.blikshteyn@haynesboone.com.
Eugene Goryunov is a partner, IPR team lead, and co-chair of the Artificial Intelligence Practice at Haynes Boone. He is an experienced trial lawyer representing clients in complex patent matters involving technologies from AI to consumer goods, high technology, networking, and wireless telephony, medical devices, and therapeutics. Goryunov has extensive experience and regularly serves as first-chair trial counsel in post-grant review trials (IPR and PGR) on behalf of both petitioners and patent owners at the USPTO. He can be reached at eugene.goryunov@haynesboone.com.
Matthew Beck is an associate in the Intellectual Property Practice Group in Haynes Boone’s Washington, D.C. office. His practice focuses on patent disputes before the Patent Trial and Appeal Board (PTAB), patent prosecution, and patent counseling. Beck has experience in inter partes review proceedings before the USPTO. Matthew’s technical expertise includes a wide array of technologies, including video compression and encoding, artificial intelligence, networking, and computer hardware and software. He can be reached at matthew.beck@haynesboone.com.