Leveraging AI In Drug Discovery To Treat MS & Other Autoimmune Diseases
By AmbreƩn Zaman Riaz, MBBS, MPA, Uppshot Lifesciences
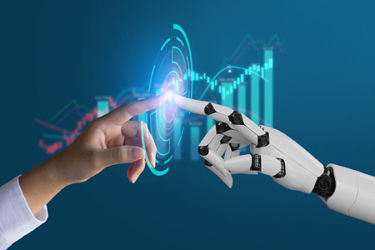
On a cold morning, a young woman walked into my office with a history of rather vague symptoms that she described as “all over the place,” including vision problems, fatigue, numbness, and weakness of the lower extremities. As a general practitioner, I could foresee the diagnosis looming before the bloodwork returned. Emma was diagnosed with multiple sclerosis (MS).
After trauma, MS is the most common neurological disease in young adults, affecting 1 per 1,000 individuals.1 More than 275 people are diagnosed with MS daily across the globe, yet a cure is still not on the horizon.
Research into the causes and treatment of MS is ongoing, and there have been several recent breakthroughs in understanding the disease and developing new therapies. One area that prominently stands out is artificial intelligence, particularly for creating personalized treatment plans for patients who may not respond well to individual drug regimens.
The advancement of machine learning algorithms means we can use large pools of genetic data to identify potential risk factors and disease-causing genes. As of 2021, using machine learning algorithms to analyze large data sets of genomic information to identify patterns and associations between specific genetic variations and the development or progression of MS has become mainstream. Pharmaceutical companies can also use AI to identify which drugs will likely be most effective for a particular patient based on their genetic profile. AI is bridging the gaping schisms in autoimmune disease treatment options despite opposition from those who believe that AI can pose risks, especially in terms of privacy.
Artificial intelligence is now being touted as a panacea for previously unanswered questions regarding MS because it provides a window into developing new drugs for the underlying genetic causes. By analyzing genetic and biological information that was previously unavailable, researchers can modify and create new drug targets and develop more effective treatment options that cover a plethora of neurological diseases, not just one specific autoimmune phenomenon. For instance, Biogen and Eisai are collaborating to create an AI-based drug discovery platform for neurological illnesses, including MS.
Machine learning algorithms are carefully interjected into the data sets to predict how different drugs will interact with the body. Thus, they could help to identify potential side effects or drug interactions, reduce lead times of drug approval, and significantly improve efficacy.
Alongside these discoveries, there are other ways AI can provide valuable insights into the underlying biological mechanisms that contribute to the development of chronic symptoms of the disease by identifying the relevant features that are most important in predicting the MS phenotype. These insights can include genetic variants, gene expression levels, and other molecular markers. Feature selection algorithms such as PCA and t-SNE can be used to identify and tease out the most informative features of genetic changes that eventually lead to MS.
Data Analysis For Drug Development
During my consulting experience, I have seen that a three-pronged approach is valuable in analyzing data for personalized treatments of chronic diseases. I summarize my three-step methodology as follows:
- Model Selection: Choose an AI model that suits your research question and data set. For example, a deep neural network (DNN) can be used for predicting the MS phenotype from genetic variants or gene expression data. You can use a supervised learning model such as random forest or SVM, or an unsupervised learning model, such as clustering and association rule mining.
- Training the Model: The next step is to train the selected model on the preprocessed data. This approach involves feeding the data into the model and adjusting the model parameters to fit the data.
- Model Evaluation: After training the model, you need to evaluate its performance on a separate set of data (test data) to ensure that the model is generalizable and can accurately predict new data. Standard evaluation metrics include accuracy, precision, recall, and F1 score.
Furthermore, feature selection algorithms like PCA and t-SNE work to identify the most informative features by reducing the dimensionality of the data. In other words, they recognize the most critical variables that contribute to the outcome of interest. PCA (principal component analysis) is a linear method that transforms the data into a new set of uncorrelated variables and captures the maximum amount of variance in the data. t-SNE (t-distributed stochastic neighbor embedding) is a non-linear method that maps the data onto a lower-dimensional space while preserving the local structure of the data points. Both methods are commonly used in genomics research to identify the most informative features.
Pitfalls Of Using AI In Drug Discovery And Preclinical Development
Although the potential to revolutionize treatment options for MS with AI models is genuinely significant, considerable challenges must be carefully considered and addressed. For instance, limited availability and quality of data can negatively impact the outcome of the drug evaluation process. In the case of MS, there may be constraints on acquiring high-quality data due to the complexity of the disease and the variability of patient responses. Moreover, the data may be biased or incomplete, leading to inaccurate or unreliable results.
AI models can be complex and opaque, making it difficult to understand how a model arrived at its predictions or recommendations. This conundrum can be misleading in drug discovery, particularly for autoimmune diseases such as MS, where decisions must be made based on interpreting complex biological systems. Therefore, ensuring that the AI models used in drug discovery are transparent and interpretable and that the results can be validated through independent experimental methods is crucial.
AI models may perform well on a particular data set for multiple sclerosis but may not generalize to new data sets or populations. This information is especially relevant in MS drug discovery, where patient heterogeneity and disease variability may affect the generalizability of the results.
The ethical frameworks around AI drug discovery raise essential considerations, such as the potential for biased or discriminatory algorithms, privacy concerns, and the use of sensitive health data. It is vital to address these ethical considerations and ensure that AI is used responsibly and ethically.
AI For Silico Modeling
More recently, AI has been used to develop in silico models of drug interactions with biological systems. These models use data on the drug's chemical properties, target protein structure, and other relevant factors to predict how the medicine will interact with the body at a molecular level — leading to insights into the drug's efficacy, toxicity, and other essential properties.
Undoubtedly, there is tremendous potential for AI tools to predict how drugs will interact with the body by analyzing large amounts of data and identifying patterns and relationships between drugs and biological systems, yet there are drawbacks, such as lack of transparency, that are raising eyebrows in the pharmaceutical industry. Many researchers think of AI algorithms as "black boxes" because their decision-making processes are not transparent to human users.
Considerable resources are being poured into developing pharmacokinetic models that predict how a drug will be absorbed, distributed, metabolized, and eliminated in the body. These models can consider factors such as drug dose, route of administration, and patient characteristics to predict drug concentrations in different tissues and organs.
Paradoxically, AI can also predict the toxicity of drugs by analyzing molecular structures and identifying potential toxicity risks. It can help to identify drugs that may cause adverse effects and prioritize drugs that are less likely to cause harm. We can now predict how MS drugs may interact with other medications a patient takes before placebo-controlled double-blind studies. AI can identify potential interactions and predict their severity by analyzing drug-drug interaction databases and patient data.
References
- Bert A. ’t Hart, Antonio Luchicchi, Geert J Schenk, Joep Killestein, Jeroen J.G. Geurt. Multiple sclerosis and drug discovery: A work of translation, EBioMedicine; 2021 Jun; 68:103392. doi: 10.1016/j.ebiom.2021.103392. https://pubmed.ncbi.nlm.nih.gov/34044219.
- Marco Meglio: Applying Artificial Intelligence to Monitor Multiple Sclerosis Disease Progression; Neurology Live; March 15, 2023. https://www.neurologylive.com/view/applying-artificial-intelligence-monitor-multiple-sclerosis-disease-progression.
- Dolgin E. Therapies: progressive steps. Nature. 2016; 540: S7-S9
About The Author:
Ambreén Zaman Riaz, MBBS, MPA, is a physician with an MPA from Harvard University. Her passion is solving problems in clinical medicine using AI and medical devices. She has global experience working as a healthcare consultant and social impact entrepreneur across multiple geographies.