Key Considerations For Cell & Gene Therapy Developers To Tap Into AI
By Yoshio Hagiwara, Paolo Siciliano, and Willem Van Asperen, PA Consulting
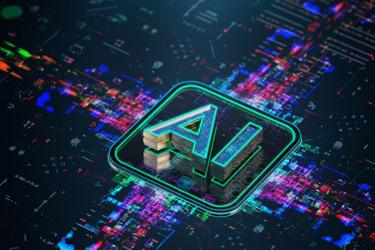
It is undeniable that artificial intelligence (AI) and machine learning (ML) are going to play a key role in the cell & gene therapy (CGT) space, as we are currently witnessing in the broader pharmaceutical sector, and beyond. As discussed in our previous article, Opportunities For AI To Assist Cell & Gene Therapy Companies, AI and ML can find a number of applications in the CGT sector, from facilitating target discovery and patient recruitment in clinical trials to optimizing manufacturing and supply chain. However, AI/ML is still an emerging field, and while it is currently one of the most talked about topics in boardrooms across the globe, around 90% of all AI/ML models never make it into production to create real business value. This is due to various factors including data-related challenges and lack of algorithm model management and handling practices. In addition, AI must be responsible, ethical, and geared toward patient safety, while retaining human oversight. CGT developers interested in leveraging AI technologies need to be aware of such aspects as well and take into account a number of strategic considerations before adopting AI/ML technologies within their organizations.
Challenges Associated With Developing AI Solutions In The CGT Space
To deliver meaningful and measurable impact from AI solutions, therapy developers and key players across the CGT ecosystem need to carefully consider and address several challenges associated with data and regulations. Here we briefly describe some of the challenges organizations are likely to face when trying to implement AI solutions:
- Limited data availability and data heterogeneity: In general, AI algorithms need to be trained on large amounts of good-quality data. In the healthcare space, this can pose a logistical challenge for data collection and curation, especially when it comes to rare diseases (the typical focus of many CGTs), where the amount of data is limited due to the small number of patients and studies. In addition, data associated with rare diseases is usually highly fragmented, as it likely originates from different sources, where it is stored in different formats and follows different labeling standards. Before developing AI algorithms for applications in the development and manufacturing of CGT for rare diseases, these highly fragmented data sets will need to be standardized into high-quality data to be used for algorithm training.
- Multimodal data integration: Highly accurate and sophisticated AI algorithms for CGT applications are likely to require the integration of diverse types of data (e.g., genomics, clinical, medical imaging) in order to augment the insights and information provided to the user. Integrating data of different modalities while reducing the noise could be highly complex and multidisciplinary collaboration between omics, bioinformatics, data science, and AI experts is essential to develop robust AI algorithms.
- Limitations in data access and ensuring data security: In some cases, developing AI algorithms may require access to healthcare data such as electronic health records (EHRs) and patient-generated data. The private nature of these data sets imposes additional barriers to AI-solutions developers with regard to data accessibility. A potential solution to this challenge is the use of synthetic EHRs (i.e., artificial but realistic electronic health records), which could replace or complement medical data to train AI algorithms when regular EHR data are not sufficiently available. In addition, considering the sensitivity around patient data and medical records, security measures need to be robust and adaptable to evolving threats to protect these data from breaches and unauthorized access.
- Lack of clear algorithm validation and regulatory hurdles: Once an algorithm model has been developed, the next big challenge is how this model is going to be validated to ensure accuracy. This is more crucial for certain post-development stages where AI solutions may replace critical human-performed steps and tasks (e.g., quality control and product release). In such use cases, there is low tolerance for error, as the accuracy in these steps is directly linked to patient safety. This is likely to attract greater regulatory scrutiny, presenting additional hurdles and uncertainty to AI implementation.
Adopting AI Requires A Robust Strategy In Place
For CGT developers, there are strategic considerations that need to be carefully taken into account before adopting AI/ML technologies. Here are some tips on how CGT players can successfully adopt AI/ML tools to create business value.
Development Of AI Solutions: Outsourcing Vs. In-House Development
Once CGT developers or manufacturers have defined which of their internal challenges can be addressed by AI/ML solutions, the first decision is whether to develop and/or integrate such technology internally or through a third-party expert. Development of AI through external partnership is the most straightforward approach in the short term, as it minimizes both cost and risk compared to in-house development. However, this does not allow organizations to internalize such expertise, which could then be transferable to other R&D areas, leading to the need to rely on external expertise whenever required in the future, increasing cost in the long term. Additionally, when outsourcing the development of AI solutions, it is critical for CGT organizations to ensure that their background IP and any data used for algorithm development, update, or refinement remain with the organization and not with the outsourcing partner. An additional risk of completely relying on an external partner for the development of AI solutions is that the pace of change in how AI use cases are being developed and deployed means that AI leaders today may not be leaders in the future. Ensuring that deployed solutions can be easily changed is essential, and it is important for CGT developers to keep monitoring the AI industry and stay up to date with the key players in the sector.
On the other hand, in-house development can provide CGT organizations with the optimal AI solution to their challenges, i.e., solutions that are bespoke and strategically aligned with the company’s broader needs. However, in-house development requires the internalization of skills that are commonly not part of a CGT company’s core capabilities, hence leading to longer time frames and higher costs in the short term.
An alternative option to the above is to adopt a hybrid approach, which is usually an attractive solution for many biotech companies. This involves developing the core capability internally, while supplementing it through collaboration with external providers to take the best of two approaches. For example, a third party can be contracted to provide a solution for the short-term problems, while an internal team is built and tasked to develop solutions of higher value to address longer-term challenges.
Appropriate Data Acquisition And Handling Strategies Are Vital For Successful AI/ML Development
Data is fundamental for AI development. Having a proper data governance and data strategy in place to cover activities such as data acquisition, data storage, and data security is essential to ensure the effective and efficient development of AI solutions within an organization.
First, data has to be FAIR (findable, accessible, interoperable, and reproducible) so that the largest amount of data can be processed and analyzed. Data capture via best practices (such as electronic batch records/electronic lab notebooks) allows data to be captured and utilized as best as possible and can enable CGT companies to avoid the classic “garbage in, garbage out.”
Unstructured data is quite common in early R&D and is a key challenge that data scientists face, hindering data analysis, integration, and interoperability and, ultimately, the development of accurate and reliable data analytics tools and algorithms. Regardless of sources and provenance, data must be processed and converted into a structured format.
In addition to data quality and data structure, it is essential to ensure that proper security measures are correctly in place so that data and IP are protected. For organizations working in the CGT space (and for therapy developers in general), access to personal health data is usually necessary. Having robust data privacy protections in place, in line with the local regulations (such as HIPAA and HITECH in the U.S. and GDPR in Europe), is essential before acquiring personal health data sets.
A Process That Covers And Manage AI/ML Algorithms Throughout Their Life Cycle Needs To Be In Place
When developing AI solutions, a complete life cycle process for procuring data, developing, and/or deploying AI needs to be in place. The AI algorithm model life cycle can be divided into three phases:
- Think Big: Initiation/Ideation Stage
The Think Big phase is all about envisioning how data can support purpose and strategy for future business growth. Results of this phase can be disruptive business and data concepts or ideas to build advocacy for the data-driven organization.
- Start Small: Algorithm Development Stage
The Start Small stage involves developing the algorithm and includes steps such as data collection, data wrangling, feature extraction, model selection/training, and model testing and validation. Each of these steps has potential risks (such as errors in data items during data collection). It is vital that data engineers and data scientists work together to identify such risks and create mitigation plans. Most data science projects will stop at the Start Small stage (i.e., algorithm development stage) and only a few successful ones will enter the Scale Fast stage (i.e., algorithm deployment stage), due to various factors such as difficulty in collecting the right amount of data of good quality, complexity of developing models, lack of skillsets for algorithm productization, or not meeting data compliance.
- Scale Fast: Algorithm Productization And Deployment Stage
The Scale Fast stage is about productizing the algorithm, monitoring its production, and optimizing it based on feedback. In this stage, both data engineers and data scientists need to collaborate with graphic user interface (GUI) designers to develop a user-friendly GUI for end users to understand the output and to interact with. It should be noted that ML models that make it into production can still fail due to changes in data used to train the model over time (e.g., change in demographics if such data was used), rendering the algorithm less accurate. Failures during early development or post-production stages generally occur due to barriers between development and production processes and lack of collaboration between data scientists and ML engineers.
Machine learning operations (MLOps) are a set of practices that were devised to remove such barriers and streamline the processes and manage ML models throughout their life cycles via seamless collaboration between data scientists and ML engineers. However, implementing MLOps is never as simple as buying a tool from a software vendor, as it often requires an organization-wide transformation, including implementing changes in business operation and governance. Implementation of MLOps requires collaboration among a wide range of stakeholders, SMEs, data engineers, data scientists, cloud architect, etc., as well as appropriate management and governance for each step from designing a project to algorithm production and retirement. Successful adoption and implementation of MLOps can ensure faster model development, deployment, and quality maintenance and improvement.
Ensure Ethics With Responsible AI
AI ethics are a set of principles and techniques employed to guide the moral conduct of the development and use of AI technologies. The following questions should be addressed to achieve AI ethics:
- Is the solution legitimate? Use of AI should be legitimate — teams must ensure they have a lawful basis for using data for the intended outcome and be able to justify why AI is being used in a given scenario as opposed to human decision-making.
- Is the solution fair? Input data must be representative, relevant, and accurate. Architectures/methodology should not include variables, features, processes, or analyses that are unexplainable, objectionable, or unjustifiable. In addition, outputs should not have discriminatory or inequitable impacts.
- Is the solution without bias? Development of AI/ML should actively avoid and mitigate biases throughout the development life cycle — bias can affect the outcomes of AI decision-making such that unfair outcomes are developed, potentially without any conscious action being taken to do so.
Develop An IP Strategy
When an AI/ML algorithm has been developed internally, it is crucial to protect the ownership and usage exclusivity of the algorithm in order to maintain the business value achieved. Algorithm-related assets can be protected through a combination of patent, copyright, contract, and trademark. Algorithm owners should identify IP assets and business value and determine the best option (or combination of options) to protect the assets. For example, patent registration is a powerful tool to protect AI-related assets. However, patent alone is not a complete solution. A complied, curated data set used for algorithm training cannot be patented. In this case, trade secret and copyright can be used to protect the data set. In addition, algorithm codes alone cannot be patented as they can be considered as an abstract idea or part of the law of nature, which are unpatentable; however, these can be protected with copyright. While there is no single silver bullet to protect all AI-related assets, CGT developers can secure their AI-driven business values with a robust IP strategy and combination of IP protections in place.
An AI Validation Strategy Needs To Be In Place
Model performance and quality assurance are essential for the application of an AI model. Acceptance criteria, tolerance for error, and activities required for validation may differ depending on the nature of the AI solution and its intended use. For example, use cases with no involvement of human operators in decision-making and higher risk for patient safety will require stricter acceptance criteria and lower tolerance for error. Therefore, organizations need to develop a validation strategy based on such parameters to ensure good performance and safety. For example, the International Society for Pharmaceutical Engineering (ISPE) has developed a framework for risk assessment and required validation activities. This framework takes into account autonomy degree (ranging from a fixed algorithm with no self-learning to a fully automated, self-learning model) and control design (ranging from a system used in parallel with an existing validated process/system to an automatically operating system that updates and controls itself).
Data-driven analytics and AI technologies are poised to address challenges faced by the CGT sector. Although adoption of AI in this sector is still in the nascent stage, it will offer great potential and opportunities if careful and well-planned strategies are implemented. Adoption of AI/ML tools is not merely technological in nature but also requires robust processes, operations, and governance to be in place. Therefore, businesses need to invest time to understand AI-related opportunities and challenges, prioritize business needs, and acknowledge gaps in internal skillsets before attempting to adopt this technology.
About The Authors:
Yoshio Hagiwara is a life sciences expert at PA Consulting. He has worked with and supported a number of pharma and medtech companies in technology innovation and development. His main areas of expertise include the application of data analytics and digital technologies in healthcare. Yoshio has a MSc in drug discovery and a Ph.D. in biochemistry.
Paolo Siciliano is an associate partner and life sciences expert at PA Consulting and leads PA Consulting’s work in CGT globally. He has several years of experience in supporting major pharma, biotech, and medtech companies to identify, develop, and leverage new technologies to solve business needs, as well as improve their innovation and product development processes. His main areas of expertise range from technology and commercial strategy to technology development, across a number of therapeutic areas. Paolo obtained a Ph.D. in molecular biology and worked as a senior research scientist in biotech companies in the U.K.
Willem van Asperen is the chief data scientist at PA Consulting. He has more than 20 years of experience in solving business challenges by applying a broad range of software engineering, data analytics, and artificial intelligence (AI) techniques. With a background in computer science and specializing in machine learning (ML), robotics, and neural networks, van Asperen develops predictive models for clients across a range of sectors, including healthcare and life science, public services, finance, and energy & utilities. He leads PA Consulting’s data science capability across multiple geographies and is an executive lecturer at Nyenrode Business Universiteit.