6 Drug Discovery Learnings From The Lab
By Diane Hollenbaugh, Ph.D., chief scientific officer, Bonum Therapeutics
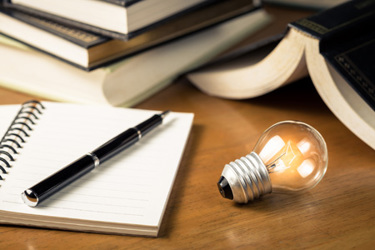
Experiments big and small are part and parcel of drug discovery. The common element to all of these centers on how we think about what we are doing, how we problem solve, and how we interpret and apply the things we learn. In this article, I’d like to focus on several insights from the perspective of bench science that pertain to experimental design and data generation. These concepts apply not only to individual experiments but also more broadly to considerations regarding an experimental path, a field of endeavor, or even to building a company.
Everything we do is constructed on a foundation of data. That foundation can be weak or strong and can be assembled in a lot of different ways, being mindful that how you build your foundation will affect what you can create on top of it. Data isn’t just the elements on a graph – it’s something that encompasses all of the features that went into its generation and all of the ways you think about interpreting it. Considering these elements -- and not just how you get to a useful data visualization -- will allow you to better turn data into real knowledge.
1. If You Can’t Describe An Experiment’s Possible Results, Rethink It.
Some experiments are fishing trips, the “hypothesis generating” sort where you perform them to see what happens. And some experiments are carefully designed to answer a very specific question. To get useful answers, you need to know what you are looking for before you begin. Perform the experiment virtually and consider all the possible outcomes before you do the work in reality. If you can’t learn something from each of the possible outcomes, you need to go back and understand why you can’t. That will help you change the experiment or, perhaps, drop it altogether. It’s not just a matter of ultimately generating useful data, it’s also a matter of efficiency in both time and money. Do the experiment in your head before you perform it at the bench.
2. Never Skip Or Explain Away The Controls — Ever!
This should seem obvious, but it isn’t always. Too often, I have seen a researcher make the decision to ignore their control results because they didn’t fit what they were expecting to happen. Sometimes that simply leads to wasted time and effort, but in the worst cases, dismissing the controls can result in worthless data and wrong scientific conclusions with implications not only for your work and career, but potentially the work of others. If you find yourself struggling to comprehend your control results, don’t fight it – work to understand it! There is information there that is important and useful, everything from a reagent that isn’t what you think it is to the realization that the general concept of your experiment is wrong. Skipping or ignoring the controls is a big pothole. Don’t fall into it.
3. Know Your Reagents.
The late American television chef Julia Child used to say that if you don’t have the exact ingredient that a recipe calls for, it doesn’t mean you can’t make that dish. However, if your substitute is an inappropriate or poor quality one, you will get a bad result. The same goes for experimental reagents. If you spend all of your time wanting to have the perfect reagent, that perfection may be much more than you need. However, if your proteins are bad, you can’t trust results you get from them. Your quality control should be appropriate to the answer you’re looking for. Know what you have and what the limitations of those reagents are, and if they still can answer a question, they might still be useful. Perfection can be the enemy of “done.”
4. Use The Right Tool For The Job.
New technologies and research tools are exciting, and it can be tempting to want to use them. But while science and technology go hand-in-hand, they don’t always advance synchronously. Sometimes the amazing new technology you’d like to use is not really applicable to your research problem. Sometimes the tool doesn’t exist to perform the experiment the way you want, and you need to choose another way to answer your research question or find a way to make the tool you need. Moreover, sometimes a well-proven, old-school method is the most useful one for your experiment. Just because a technology or tool is old doesn’t mean it is not the right tool for the job.
5. Memory Is Short. Record The Details.
Here is an experiment to perform – go back to your notebook and read about an experiment you did a year ago. Can you tell for sure what you did? Could someone else? It is so easy to fall into shorthand. After all, you are deep in the details and know what the shorthand means. But will you a year from now? Writing it down includes noting why you did it, and all the things you were thinking about it at the time, and what you believe you should do next. Your interpretations are valuable, so record them in real time. A later experiment can lead to revisiting and reinterpreting an earlier result, and you don’t want to miss anything. And since the outcome of an experiment is not just a graph, but all the information that went into creating it, if you lose that information the data is compromised and the value of it is lost.
6. Structured Thinking Can Free Creative Thinking.
Do you remember your first lab class and how torturous it was to write up the experiment in your lab notebook? You had to write out all of the sections – your hypothesis, methods, data, and conclusions, even for something as simple as measuring the temperature of boiling water. It may have seemed silly at the time; I know it did to me. But what we were learning was a toolkit for the scientific method. It is a way to systematically ask yourself, “What do I think I know and what am I trying to figure out here?” We don’t always have to write the entire process out, but using it is an essential part of a “back to basics” strategy that has survived for centuries because it works.
Going back to first principles is the second essential part of this strategy. Sometimes when people are faced with an experimental or scientific problem, they forget to ask themselves, “Does this make sense based on what I absolutely know to be true and what I believe to be true?” Getting back to first principles allows you to effectively question what you are doing, while the scientific method provides a structured way to remove yourself from tunnel vision. Together, these concepts provide a way to see things you might have missed and free you to think about things in a different way. Ultimately, it is about being systematic, rigorous, and structured in how we think about and do things, which frees us to be creative. We can see things for what they really are, not just what we thought them to be.
While these six learnings may seem simplistic, it is precisely for that reason we might overlook them. Once they are habits, it is still worth checking in on them. They keep your data foundation strong and free you to think beyond what is immediately in front of you. That is where good science emerges!
About The Author:
Diane Hollenbaugh is a drug discovery scientist and chief scientific officer of Bonum Therapeutics (formerly Good Therapeutics). She has more than 25 years of experience in biologicals research, research management, and discovery and early development with a focus on immunology and oncology. Previously, she was executive director, immuno-oncology discovery at AbbVie, where she oversaw the growth of IO efforts, including early discovery and translational science, licensing partnerships, and five discovery projects brought to IND. Prior to AbbVie, she has experience at large and small companies, including BMS, Medarex, FivePrime Therapeutics, and Schering-Plough/Merck. She received a B.S. in chemistry from the University of Washington and a Ph.D. from the California Institute of Technology Division of Chemistry and Chemical Engineering, and completed her postdoc training at the Bristol-Myers Squibb Pharmaceutical Research Institute. She is an inventor on more than 20 issued patents.