ChatGPT-4: Practical Applications For The Biopharma Laboratory
By Tim Sandle, Ph.D.
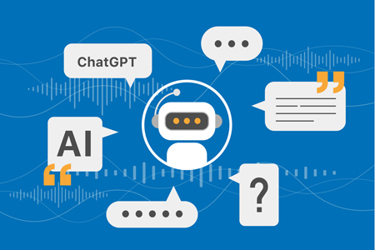
Artificial intelligence (AI) has significantly improved in recent years, and several aspects of AI have made their way into the pharmaceutical and healthcare sectors. One such area is in the laboratory. In particular, large language models (LLM) such as chat generative pre-trained transformer (ChatGPT) are reaching a level of maturity. Laboratory managers need to understand the capabilities of advanced AI tools and other breakthrough innovations. Such an understanding will allow personnel to recognize the opportunities and threats that emerging technologies can deliver.
In this article, three examples of how Chat GPT-4 can aid laboratories are presented together with some of the technological, regulatory and ethical limitations.
Why ChatGPT-4?
The most common AI-driven tool is ChatGPT. This is a natural language processing model developed by OpenAI (now part of Microsoft). The current iteration, GPT-4, was released in March 2023. There are other LLMs available; however, Chat GPT-4 has 180 million users worldwide and this level of interaction results in this form of AI being the most likely to be used within the laboratory environment.
ChatGPT deploys a neural transformer network to process and generate text independently. This includes using the functionality built into internet browsers like Microsoft Edge to answer users’ questions and interact with them through “natural language” (the “chat” aspect). To improve accuracy, reinforcement learning from human feedback (RLHP) is conducted, where human graders rank GPT responses to a set of queries.1
It is probable that GPT-4 will be eclipsed by more powerful AI entities. In the meantime, ChatGPT-4 is a powerful electronic brain, capable of extracting meaning and patterns from massive amounts of scientific data.
Simplifying Research
Chat GPT-4 is capable of “reading” and interpreting science texts and responding to user commands. This provides it with considerable potential as a research tool. On a general level, the user can provide a general instruction for a specific task, such as “Read and summarize this article on pharmaceutical compounding” or something more specific, such as “Read and summarize the current FDA guidance on biosimilars.” Commands can be re-run for researchers who crave more up-to-date and accessible insights into a given area of study.
Such requests can be made in any language and be applied to papers, reports, spreadsheets, patents, and complex statistical formulations.2
Supporting New Drug Development
A 2024 study from the U.S. National Science Foundation has helped to establish the application of AI to increase the speed and volume of scientific discoveries. This led to the development of a series of software modules called Coscientist that are driven by ChatGPT-4. These modules can perform the tasks that research scientists undertake, such as searching for information about chemical compounds, assessing technical manuals, generating computer code for experiments, and analyzing the resulting data. The difference is that LLMs like Coscientist can do this faster and probably more accurately.
As detailed in a paper published in Nature,3 scientists evaluated Coscientist’s performance of "chemical reasoning," which they define as the ability to use chemistry-related information together with previously acquired knowledge to guide one's actions. Coscientist was able to take data stored in a simplified molecular input line entry system (SMILES) format (a type of machine-readable notation representing the chemical structure of molecules) and provide the process for synthesizing aspirin, acetaminophen, and ibuprofen. The AI also was able to program robotic laboratory equipment for the synthesizing method.
The paper also demonstrated how tools like ChatGPT can improve the replicability and reliability of experimental results.
Image Assessment
One feature introduced in GPT-4, not present in earlier versions, is the ability to analyze images. This presents the potential to help doctors make diagnoses, for microbiologists and pathologists to assess cultures, and to assist with more general assessments of laboratory data sets. For example, when assessing a new compound, graph AI techniques can integrate data, including laboratory test results and imaging data. By creating a network of heterogeneous data points, patterns and correlations between disparate pieces of information can be discerned.
Other LLMs specifically tailored for assessing microscopic images have seen even greater success. This includes powerful image generators and interpreters such as Midjourney and Stable Diffusion.
Other Applications
Some other applications of LLMs in the laboratory setting include:4–6
- Education: For new entrants to the laboratory (as well as those undergoing studying), LLMs provide a means to improve learning.
- Note taking: ChatGPT-4 can aid laboratory personnel in taking notes and minutes and provide real-time suggestions. GPT-4 can create notes in familiar formats.
- Translation: LLMs can translate texts in languages that are unfamiliar and promote greater international collaboration.
Limitations
Limitations of LLMS include their technological immaturity and the extra care necessary when applied within a GxP setting.
Technological Limitations
All LLMs are limited by their training data, and this may or may not include all of the technical areas required for a given application.7 It also stands that all forms of AI contain the biases of their development. This may arise when GPT-4 struggles to understand the context of a conversation, leading to misconceptions or incorrect responses.
Another concern is with accuracy because, sometimes, depending on the information sources used, ChatGPT’s capabilities can lead to fabricating facts (a false response by GPT-4 is known as a “hallucination”8). Similarly, where policies are frequently revised, comments provided by GPT-4 algorithms may be out of date or incorrect. It also arises that when comparisons are presented, the reasoning deployed is weak. Moreover, ChatGPT has no long-term memory.9
Philosophically, what constitutes acceptable overall AI performance still needs to be answered.
GxP Concerns
Care is required when ChatGPT-4 and other systems are used in GxP environments. Under no circumstances should LLMs be used for completion of batch records. It is imperative that these are completed contemporaneously by the person who performed the task, using measured data.
If tools like ChatGPT-4 are to be used to generate procedures, or rather assist in their generation, these need to be sense checked by competent persons and subject matter experts. While there can be advantages to using language simplification to make instructions clearer, critical steps and compliance considerations may be missed or misunderstood by the LLM.
The U.S. FDA10 and the European Union11 currently have complex regulatory frameworks for digital technologies, especially in the health diagnostics space. Many of the concerns expressed are applicable to pharmaceuticals, especially the need for final human determination. Regulatory opinion may shift as the LLMs become more sophisticated.
Ethical Concerns
There are some general ethical concerns with AI, including the reliance placed on its decisions and the level of autonomy afforded. There are also privacy issues, including retaining data collected, and GPT-4 users should be aware of such potential privacy concerns. Fraud is another example, for it is possible for ChatGPT to produce a convincing scientific abstract based on imaginary research data to the degree that this will be difficult to detect.12
Care also needs to be taken when considering the limitations of LLMs in the pharmaceutical environment to avoid any situation that might lead to patient harm. AI needs to follow informed rules and policies to prevent harmful outcomes, whether intentional or accidental.
Next Steps
At its current stage, ChatGPT is best used as a supportive tool and not as a complete solution. It is prudent that all work and information provided is verified by a competent person.
References
- Thirunavukarasu AJ et al. Large language models in medicine. Nat Med 2023;29:1930-40
- Waisberg E, et al. Challenges of artificial intelligence in space medicine. Space: Sci Technol. 2022:2022
- Boiko, D. et al. Autonomous chemical research with large language models. Nature, 2023; 624 (7992): 570 DOI: 10.1038/s41586-023-06792-0
- Kung TH, et al. Performance of ChatGPT on USMLE: Potential for AI-assisted medical education using large language models. PLOS Digit Health. 2023;2(2):e0000198
- Chen TJ. ChatGPT and other artificial intelligence applications speed up scientific writing. J Chin Med Assoc. 2023;86:351–3
- Beam AL, et al. Artificial Intelligence in Medicine. N Engl J Med. 2023;388(13):1220–1221
- Lee P, et al. Benefits, limits, and risks of GPT-4 as an AI chatbot for medicine. N Engl J Med. 2023;388(13):1233–1239
- Corbelle JG, et al. Dealing with hallucination and omission in neural Natural Language Generation: a use case on meteorology. In: Proceedings and Abstracts of the 15th International Conference on Natural Language Generation, 2022. Waterville, ME: Arria, 202
- Ray PP. ChatGPT: a comprehensive review on background, applications, key challenges, bias, ethics, limitations and future scope. Internet of Things and Cyber-Physical Systems. 2023;3:121–54
- U.S. Food and Drug Administration. Artificial intelligence and machine learning in software as a medical device; 2021. Available at: https://www.fda.gov/medical-devices/software-medical-device-samd/artificial-intelligence-and-machine-learning-software-medical-device
- European Commission. Regulatory framework proposal on artificial intelligence; 2022. Available at: https://digital-strategy.ec.europa.eu/en/policies/regulatory-framework-ai
- Gao CA, et al. Comparing scientific abstracts generated by ChatGPT to original abstracts using an artificial intelligence output detector, plagiarism detector, and blinded human reviewers. bioRxiv. 2022:2022.12.23.521610
About The Author:
Tim Sandle, Ph.D., is a pharmaceutical professional with wide experience in microbiology and quality assurance. He is the author of more than 30 books relating to pharmaceuticals, healthcare, and life sciences, as well as over 170 peer-reviewed papers and some 500 technical articles. Sandle has presented at over 200 events and he currently works at Bio Products Laboratory Ltd. (BPL), and he is a visiting professor at the University of Manchester and University College London, as well as a consultant to the pharmaceutical industry. Visit his microbiology website at https://www.pharmamicroresources.com.