Can Molecular Modeling Overcome The Limitations Of Drug Discovery AI?
By David Kita, Ph.D., Verseon International Corporation
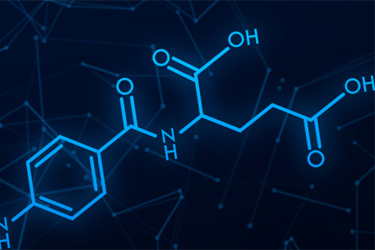
While modern medicine includes a wide range of intervention strategies — such as peptides, antibodies, biologics, and gene therapy — small molecule drug discovery is still the “brick and mortar” of the global pharmaceutical industry, driving the bulk of the industry’s $1.27 trillion annual sales revenue.1 However, designing novel, potent, and selective small molecule drugs that can be safely administered as pills or capsules with minimal side effects remains a daunting proposition. Each approved drug costs billions of dollars to develop and requires more than decade of R&D, clinical testing, and regulatory review to reach market. Along the way, the vast majority of drug candidates end up failing in clinical trials, often many years and hundreds of millions of dollars downstream.
Drug discovery has greatly benefited from the bioinformatics revolution that began with the Human Genome Project and the concomitant genomics renaissance of the 1990s. A great deal of effort and resources have been devoted to acquiring large biological data sets and gleaning actionable insights from the many genomes, proteomes, cellular mechanisms, metabolic pathways, and various biological networks of humans and other species.
But our progressively improving comprehension of human biology shows no signs of resolving the foremost obstacle in small molecule drug discovery: the efficient design of truly novel drugs. For the last decade and a half there has been a relative dearth of truly novel small molecule drugs receiving regulatory approval. To mitigate the costly risks of failure, most small molecule drug candidates currently submitted for approval are chemically similar to existing approved drugs and share similar pharmacological profiles. The result is a proliferation of so-called “me-too” drugs. If there is any field ripe for innovation, it is small molecule drug discovery. Perhaps, then, it is no surprise that the application of AI in drug discovery has captivated the interest of many companies and investors.
Limitations Of AI In Small Molecule Drug Discovery
In his April 27 column titled “Small Molecule Drug Discovery: Can AI Do It All?”,2 my colleague Dr. Anirban Datta described several major obstacles to using AI to find new drug candidates that do not resemble previously known chemical matter. First and foremost is the so-called “data problem.” It is no secret that AI-based small molecule drug discovery requires an overwhelming amount of data. However, current experimental methods like high-throughput screening or calorimetry used to assess the binding of a small molecule (a “ligand”) with a disease-associated protein require that the compound first be synthesized. Unfortunately, unavoidable synthesis bottlenecks strongly limit the size and diversity of compound collections for experiments. The lack of diversity is so pervasive that despite decades of synthesis efforts and several hundred million compounds generated, the estimated total number of chemically distinct compounds found across all corporate compound collections is less than 10 million. This pales in comparison to an estimated 1033 (or a billion trillion, trillion) distinct, drug-like compounds that are feasible to make under the rules of organic synthesis.3 For the foreseeable future, there will simply not be enough experimental binding data available for AI models to reliably predict truly novel, potent, and selective small molecule drug candidates. Consequently, untold numbers of promising small molecule treatments remain beyond AI’s current reach.
Several additional aspects of protein-ligand binding further exacerbate this data problem.
First, the required amount of training data for successful learning scales exponentially with the dimensionality of the feature space, the so-called “curse of dimensionality.”4 Unfortunately, due to the complex nature of protein-ligand binding, the dimensionality of the feature space used in an AI model is by necessity extremely high — certainly much higher than that of a protein or ligand alone. Moreover, the combined feature space of a protein-ligand system is much more than just that of the protein and ligand simply concatenated together because it must also represent complex relationships between the protein and ligand during binding, including intermolecular and solvent contacts, as well as relative conformational, translational, and rotational variables. Consequently, the enormous amount of data required to successfully train an AI model for reliable prediction of truly novel small molecule drug candidates may not be possible to achieve via conventional, real-world experiments.
Second, protein-ligand binding is highly sensitive to small perturbations in either the chemical structure of the ligand or the three-dimensional coordinates of the bound protein-ligand complex (“binding mode”). Both types of perturbations can lead to large variations in the likelihood or strength of binding (“binding affinity” or “binding free energy”) and hence sharp transitions in the feature space. Such sharp transitions are notoriously hard to successfully interpolate unless there is much more densely packed data available for training, thereby placing even higher demands on the amount of data required for successful learning.
Third, the lack of chemical diversity represented in experimental data sets is a serious liability for AI attempts to predict truly novel small molecule drug candidates. Existing experimental binding data available to train AI models cover only an extremely narrow subset of synthetically feasible drug-like compounds, making it extremely challenging for an AI model to extrapolate to truly novel small molecule binders via training on labeled data sets (i.e., supervised learning). An AI-only platform is almost certainly doomed to interpolate within the tiny portion of chemical space covered by experimental data sets, likely predicting small molecule binders that will have high chemical similarity to already known synthesized molecules.
Can Molecular Modeling Overcome AI’s Data Problem?
First, let’s discuss the concept of molecular modeling.
Molecular modeling harnesses the rules of quantum and/or classical physics to simulate and analyze interacting molecular entities. Protein-ligand binding is a highly challenging molecular modeling problem. Thousands of protein atoms interact with one another and those of the ligand via complex molecular mechanisms while immersed in water. Both the protein and the ligand are flexible and can adopt different conformations as they interact, because many chemical bonds in both the protein and ligand can vibrate, bend, or twist. With the notable exception of allosteric binding, most changes in the protein’s shape are comparatively mild as it binds to a small molecule, though they cannot be ignored. In contrast, ligands can be highly flexible and can adopt a large variety of 3-D conformations for their size. Small molecules can also feature complex ionization states, tautomers, and stereoisomers.
Long before the recent surge in interest in application of AI to drug discovery, various groups sought to break the synthesis bottleneck via other computational approaches. They attempted to simulate how strongly a truly novel drug-like small molecule may — or may not — bind with a target protein prior to synthesis. Various computational approaches included ligand-based, structure-based, and fragment-based drug design. Fueled by an abundance of experimental protein structures and high-quality homology-based models, structure-based drug discovery (SBDD) attempts came to predominate over time. SBDD methods heavily rely on some form of molecular modeling, either physics-based or heuristic-based, using structural information for the target protein and a potential small molecule binder. SBDD methods date as far back as the pioneering DOCK program developed at UCSF in the 1980s. DOCK was so influential that computational methods used to predict the binding mode of protein-ligand systems are now typically referred to as “docking” methods. Computational methods to heuristically approximate the binding affinity between a protein and a ligand or to rank-order potential binding modes across a collection of small molecule binders are typically referred to as “scoring” methods.
Unlike AI, physics-based molecular modeling does not have a data problem, but it does have a “complexity problem.” While our understanding of the quantum world is quite advanced for atoms and simple molecules, a full quantum description of protein-ligand binding is not currently feasible. Despite advancements in computational physics, a full description of molecular systems grows exponentially harder as the number of atoms increases. There is insufficient computing power to solve even a single protein-ligand system via brute force sampling — whether in the quantum or the classical regime — because of the sheer number of degrees of freedom involved. This limitation has necessitated the development of more efficient computational schemes that treat protein-ligand binding as a complex optimization problem. High-powered modeling approaches such as molecular dynamics (MD) simulations, free energy perturbation (FEP), and QM/MM hybrid modeling, though perhaps computationally tractable for individual protein-ligand systems, require such a large amount of computational power that they are not extensible to large-scale virtual library screens against a target protein. Other modeling approaches either attempt to develop semi-classical approximations of quantum phenomena or employ various heuristics or simplifying approximations, trading accuracy for speed and reduced complexity in order to make molecular modeling more tractable.
For many years, in order to skirt the complexity problem, conventional docking and scoring methods typically employed simple approximations or heuristics that do not well represent the underlying molecular interactions. As a result, problems with accuracy bedeviled the field for many years. Then, in the mid-2000s, the field of molecular modeling as applied to drug discovery began to shift. Many of the more established methods that had previously employed various physics-based or heuristic approaches gravitated toward empirical-based models relying on statistical training-set driven techniques, though with mixed results. The accumulation of experimental measurements for protein-ligand systems — both in terms of bound structures and binding data — provided the motivation for this shift, though with perhaps limited utility. One could even view the recent application of machine learning as the logical extension of these earlier training set-driven, empirically based approaches.
Meanwhile, others continued to refine physics-based molecular modeling methods, resulting in significant improvements in the understanding of both the complex molecular interactions that mediate protein-ligand binding and how to better model those interactions. In just the last decade or so, great strides have been made in using physics-based molecular modeling to predict binding poses and to a lesser extent, the rank prioritization of potential binders. Indeed, the latter has proven to be a more difficult nut to fully crack, particularly when estimating binding free energy, which is a fiendishly complex physics problem. There certainly is still room for improvement in order to reduce false positives and improve computational efficiency, particularly when expanding the scope to screening billions of potential small molecules against a target protein.
It should come as no surprise that these challenges were a major motivation to investigate the use of AI and deep learning in early drug discovery. Yet despite the recent enthusiasm for AI-based drug discovery, the most favorable computational approaches for estimating the binding affinity of a truly novel protein-ligand system continue to be those that rely on physics-based molecular modeling, especially when the small molecule is not chemically similar to other compounds in experimental training sets or the protein is not well characterized. Of course, some experts expect AI to overcome such difficulties based simply on the scaling of future experimental data. However, as already discussed, the current — and foreseeable — state of experimental data, the necessity of a feature space with high complexity and dimensionality, and the weakness of deep learning in extrapolation all cast doubt on such assumptions.
Combining AI, Molecular Approaches May Be The Answer
Given the data problem of AI-based drug discovery and the complexity problem of physics-based molecular modeling, the challenge of efficiently finding drug candidates dissimilar to known clinical entities would appear to be quite daunting. However, a hybrid approach, wherein one computational scheme serves to complement and bolster the apparent weakness of the other, may be the answer. While there may be multiple ways to potentially synergize the combination of AI models and physics-based molecular modeling to improve predictive power without unduly sacrificing speed, there is one strategy that readily stands out — the use of molecular modeling to overcome the data problem of training AI models.
Of course, high-quality experimental binding data remain the gold standard for AI model training, though for a limited chemical diversity of small molecules. The problem is that there is simply no way that experimental data will provide meaningful, let alone dense, coverage of the complex feature space associated with protein-ligand binding, particularly for systems involving previously unexplored drug-like compounds. There is no doubt that experimental data are the backbone of labeled data sets for supervised learning, but it simply is not enough for the task of reliably predicting truly novel small molecule drug candidates.
The only foreseeable way to more densely sample such “unexplored” protein-ligand systems is via computation. Physics-based molecular modeling is the most reliable option available. Recent advances in physics-based molecular modeling have already proven quite robust in the accurate prediction of binding modes. Moreover, high-powered molecular modeling simulations (e.g., FEP, MD, QM/MM hybrids) are still the best available tools for the computational estimation of the binding free energy for a protein-ligand system, albeit at a steep computational cost and with room for additional improvement. The binding free energy, a complex thermodynamic quantity involving a multitude of different bound and unbound protein-small molecule states, is of particular importance, since it is the definitive physical metric to quantify the likelihood of a small molecule to bind to a protein.
In this way, advanced physics-based molecular modeling could potentially be used to generate data for novel protein-ligand systems for import into the training of an AI model, in addition to available high-quality experimental binding data for known protein-ligand systems. However, because experimental data and molecular modeling predictions are quite disparate in how they are sourced and characterized by substantially different error distributions, a straight import into labeled data sets for supervised learning may not be wise. A more useful stratagem is likely to be a combination of supervised learning on experimental binding data and reinforcement learning on data compiled via large-scale physics-based molecular modeling, where the rewards and penalties for reinforcement learning reflect the estimated binding free energy, the very same quantity that determines how strongly a small molecule and protein may bind according to the laws of physics.
Of course, there is no free lunch. The requisite amount of computation dedicated to physics-based molecular modeling would be enormous. But computational resources are easier to scale than laboratory methods, particularly when shackled by synthesis bottlenecks. Moreover, molecular modeling simulations can be run en masse offline when dynamically growing the additional data sets for reinforcement learning. Any speculation on how many such additional novel protein-ligand systems would need to be simulated in order to achieve a desired level of accuracy is beyond the scope of this article. But it would certainly be a comparatively small subset of the vast chemical universe of synthetically feasible drug-like compounds, and certainly far more attainable than waiting aimlessly for laboratory methods to catch up.
In the end, such hybrid AI models would learn based on both experimental data and high-powered molecular modeling simulations in order to efficiently screen large collections of truly novel virtual small molecules against a target protein. In essence, the learning accumulated by such hybrid AI models would circumvent the complexity problem of molecular modeling, while the use of physics-based molecular modeling to supply much-needed data for reinforcement learning would overcome the data problem of AI-only approaches.
References
- At the close of 2020. See https://www.statista.com/topics/1764/global-pharmaceutical-industry/.
- https://www.drugdiscoveryonline.com/doc/small-molecule-drug-discovery-can-ai-do-it-all-0001
- https://link.springer.com/article/10.1007/s10822-013-9672-4.
- https://towardsdatascience.com/curse-of-dimensionality-a-curse-to-machine-learning-c122ee33bfeb
About The Author:
David Kita, Ph.D., is the CSO at Verseon International Corporation. He cofounded Verseon because he realized that the real value of the genomics field is not in the genomic information itself, but rather in translating that knowledge into better treatments for people. Kita has headed Verseon’s platform development to date and has overseen the company’s seven drug programs. Among his other prior ventures, he built one of the world’s first bioinformatics platforms at Hyseq. Kita received his B.S., M.S., and Ph.D. in astrophysics from the University of Wisconsin, Madison.