Beware AI Hallucinations
By Vincenzo Gioia and Remco Jan Geukes Foppen
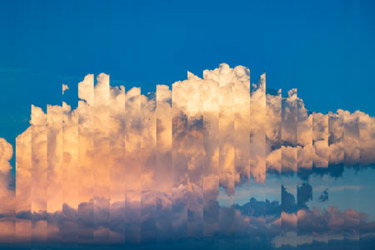
AI is becoming increasingly sophisticated, taking on responsibilities that were once exclusive to human beings. However, there are also disadvantages posed by ethical issues and the risk that AI may perform incorrect calculations. Among this type of error, the most insidious type is represented by the phenomenon of AI hallucinations, which are becoming a persistent concern. AI hallucinations refer to instances where AI systems, particularly those based on advanced models like GPT-4, generate outputs that are incorrect, misleading, or nonsensical, but are generated with a high degree of confidence. This phenomenon is called “hallucination” because it is similar to what happens when a person is under the influence of drugs or alcohol. These hallucinations can occur due to various reasons such as biases in the training data, limitations of the model's understanding, or inherent uncertainties in the problem space.
Distortions in an AI system can present themselves in various ways. Here are some examples:
- Misinterpretations: The system might misinterpret input data, leading to incorrect conclusions or inaccurate responses.
- Generation of incorrect information: The system might produce output that does not correspond to the reality of the input data or the user’s requests.
- Inconsistent responses: The system might provide responses that are not logically consistent or that contradict information previously provided.
- Unpredictable behaviors: The system might act in an unexpected or unforeseen manner, deviating from its programming or the expectations of the developers.
- Uncontrolled sensitivity: The system might not respond to certain inputs with the correct level of sensitivity, thus causing unsuitable responses.
Although the list seems long, the forms through which this problem can manifest itself are still subjects of research and experimentation.
Through the rest of this article, we’ll discuss the implications of AI hallucinations from the perspective of the pharmaceutical industry, including the challenges they present and possible solutions to mitigate their consequences.
What Are The Risks Associated With AI Models Affected By Hallucinations?
AI hallucinations are a critical issue for AI models used to make important decisions, such as medical diagnoses or financial operations. The risk associated with a hallucinated output is determined by the graded level it has on the decision-making process. Decision-making levers delegated to an AI are important because they impact the complexity of the analysis model. Delegation of the decisions would require human supervision.
How To Determine If An AI’s Processing Is Affected By Hallucinations
To date, there is no official method or tool capable of verifying whether the output generated by an AI is affected by hallucinations. Moreover, the nature of a hallucination is such that even a human operator is unable to verify its presence if the output is predictive or generative. It is reasonable to hypothesize that it is possible to identify a hallucination circumstantially by tracing its presence to a generalized inconsistency of context or to inaccuracies in the expected level of precision in the response, within limits of human control. It’s also possible to use a second AI model as a coherence check, to corroborate output validity, although this method is not considered reliable. In general, structured prompt engineering will strengthen a LLM response, but as the model is trained on the subject matter of verification, it still does not guarantee that it would detect the hallucination in all its manifestations.
Techniques For Containing The Phenomenon
There are no specific techniques for containing the phenomenon right now. From our experience, an easy way to induce hallucinations is by administering questions (called prompts) constructed in such a way as to deceive the model. For this reason, an adequate mastery of prompt engineering is mandatory for the correct use of artificial intelligence. The generation of quality and detailed prompts significantly reduces the risk of hallucinations. The inherent logic is that of techniques such as the Chain of Thought or the Tree of Thought, that is, to reduce the risk of errors by enabling the model to reason more schematically and carefully on the inputs (prompts). Another important way to detect and mitigate AI hallucinations is to adopt the approach of “human in the loop”.
What If The Problem Cannot Be Solved?
Sometimes, problems can be accepted as part of the solution. To understand this condition, one could think about the adverse reactions of drugs. What if we consider hallucinations as an acceptable business cost for the use of AI? Indeed, some companies consider hallucinations like “system crashes” and accept those events after verifying the probability of the event and the domain of knowledge.
Summary
It's been eight years since Move37 and a decade of AI-enabled drug discovery. Is it too soon to determine whether AI is delivering and making it through to approval? According to Morgan Stanley in 2022, even “modest improvements in early-stage drug development success rates enabled by the use of artificial intelligence and machine learning” could result in an additional 50 novel therapies over a 10-year period, representing a more than $50 billion opportunity. Despite the small sample size, there are promising examples of improved productivity, equal or higher success rates, and even novel targets. Even small improvements in time to market, costs, and probabilities of success have quite a positive impact on rNPV (risk-adjusted net present value). This shows that AI-generated drugs, and sometimes novel ones, can be impactful at relatively low cost and effort. At this level of investment, assets can be more easily de-risked within a portfolio, and hallucinations can be taken as an acceptable business cost. This is especially true since not all hallucinations are equally inaccurate or equally consequential (read: unpredictable behaviors and uncontrolled sensitivity). Similarly, not all novelty may be equally novel, and it would be interesting to see how attractive novel AI-generated drugs are to regulatory agencies and investors.
About The Authors:
Remco Jan Geukes Foppen is an international business executive in AI and life sciences, with proven expertise in the life science and pharma industry. He led commercial and business initiatives in image analysis, data management, bioinformatics, clinical trial data analysis using machine learning, and federated learning for a variety of companies. Foppen has a Ph.D. in biology and holds a master’s degree in chemistry, both at the University of Amsterdam.
Vincenzo Gioia is business and technology executive, with a 20-year focus on quality and precision for the commercialization of innovative tools. He specializes in artificial intelligence applied to image analysis, business intelligence, and excellence. His focus on the human element of technology applications has led to high rates of solution implementation. He holds a master’s degree from University of Salerno in political sciences and marketing.