AI/ML In Drug Discovery & Development: Potential And Challenges
By Tina Hu-Rodgers, Buchanan Ingersoll & Rooney
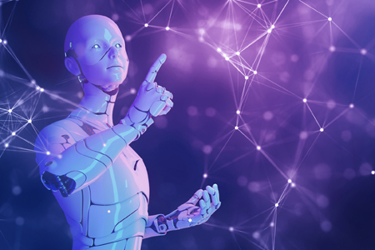
The adoption of artificial intelligence (AI) and machine learning (ML) has been one of the fastest growing trends across industries over the past decade. With the continuous advancements in technology, access to ever more powerful computers, increased availability of clinical and research data, and rapid development of novel algorithms that analyze and utilize that data, interest in applying AI and ML to healthcare, in particular, is at an all-time high.
Most of this interest, to date, has been in applying ML to improve healthcare delivery. Examples include AI/ML-assisted medical procedures;1 digital medical information management tools that use AI to improve hospital workflows and streamline patient experiences; and AI/ML-powered imaging systems and biometrics technology to aid physicians in diagnosis of medical conditions.2 Not as much public attention has been paid to the use of these technologies to facilitate drug discovery and drug development; however, AI/ML has the potential to transform these areas as well.
Traditional human-driven drug discovery and development processes are slow, extremely labor-intensive, and expensive. Because AI/ML can manage large, heterogeneous, multi-dimensional data sources, identify patterns, and predict outcomes, it has significant potential to streamline drug discovery and development and make the process much more efficient and targeted. This, in turn, will significantly reduce the time and cost required to bring better, more effective drugs to market.
However, realizing the potential of this technology will require overcoming a range of different issues, including problems with data quality and access, transparency of underlying development and validation processes, potential bias inherent in the source data as well as the algorithm’s implementation, and the lack of definitive regulatory guidance from the relevant government agencies.
The potential opportunities and associated challenges of using AI/ML in drug discovery and development are described further in the sections below.
Opportunities For Using AI/ML
The success of clinical trials depends in large part on extensive preclinical investigation and planning – i.e., identifying the most promising candidate molecules and drug targets, and then defining the investigational strategy most likely to achieve regulatory approval. Traditionally, researchers are forced to discover new drug targets through basic research, often relying on luck and trial and error to find the right one for development. As a result of this, very few drug candidates that eventually reach clinical trials actually succeed. It has been estimated that only five in 5,000 drug candidates make it through preclinical testing to human testing, and only one of those tested in humans reaches the market.3
Selecting the wrong target molecule or focusing on the wrong area of investigation can cause major delays and hinder a trial from the start, thereby wasting valuable time and resources.
ML can help researchers minimize these missteps by:
- Streamlining the process for identifying candidate molecules and drug targets and increasing the chances for success with those identified by processing massive amounts of existing research data and using predictive modeling to determine potential drug target interactions.4
- Synthesizing vast amounts of biomedical data into information that is more easily interpretable by humans.4
- Generating new, previously unknown compounds with desired biological properties (i.e., de novo drug design).5
- More effectively analyzing data to better elucidate a drug’s mechanism of action and improving chances that drugs are tested in populations most likely to benefit from them.4
A company’s ability to find better drug targets earlier in the R&D process can help move a new drug more quickly through the rest of the development process. A recent GAO report estimated that use of ML in drug discovery could allow for R&D cost savings of between $300 million and $400 million per successful drug.5
Tips For Overcoming AI/ML Challenges
Despite the potential benefits of ML approaches in drug discovery and development, these technologies are not without their challenges.
For example, in the case of de novo drug design, the particular modeling techniques used (i.e., generative adversarial networks and reinforcement learning) are prone to something called “mode collapse,” where the model only generates a small number of similar solutions.5 The ideal outcome is for the generative adversarial network to produce a wide variety of outputs.6 However, if a generator produces an especially plausible output (and, in fact, the generator is always trying to find the one output that seems most plausible), it may learn to produce only that output.6 If that should happen, then the modeling techniques utilized for generating new compounds may be artificially limiting the results produced and the full potential for innovating novel compounds with desired biological properties will not be realized. It is therefore important to test the capability of an algorithm to produce a wide variety of new structures when working with deep generative models. There has been success reported in addressing mode collapse with a novel method called “adaptive multi-adversarial training” (wherein additional data classifiers called discriminators are spawned during training); however, further work will need to be done to develop standardized approaches that can reliably overcome these types of issues in computer-aided drug design.9
In addition, if left unconstrained, generative models may produce compounds that are overly complex or impossible to produce.7 At the end of the day, a novel molecule’s predicted biological or physical properties must still be validated in the laboratory.5 Researchers must take steps to ensure that their ML-generated molecules are realistic (chemically stable and able to be synthesized) without resulting in reduced efficacy.
At a more basic level, insufficient training data and poor model calibration can lead to bias in model application. The applicability of all ML models is heavily dependent on the sources of the data used.8 This is especially impactful in the use of ML models in healthcare, where data is often plentiful but also most typically sourced from large academic referral centers in industrialized western countries (where people have better and more access to the healthcare system and therefore have a higher chance of their being used). These populations are often imbalanced in terms of disease severity and demographics, which in turn can result in similarly skewed model predictions. Skewed data can unduly influence models created with unsupervised learning methodologies, and extra scrutiny is required to determine the scope of such models. To avoid having AI and ML further exacerbate issues of bias and lack of diversity already inherent in the healthcare system, companies will need to build health equity into the algorithms they are using for drug discovery and development. Specific processes for evaluating and addressing bias in the algorithms will need to be developed and utilized routinely throughout the process.
Conclusion
There is a lot of potential for ML to improve the efficiency and quality of clinical research, but substantial challenges remain. At present, the excitement for the potential applications of ML in clinical research has outpaced its actual use.
References
- See e.g., Avenda Health’s Focal Therapy System, which is an AI-enabled lumpectomy device for in-office prostate cancer treatment; and HeartLander Surgical’s robot-facilitated heart therapy.
- See e.g., Perimeter Medical Imaging AI, which uses AI to help surgeons determine if cancer is still present after surgical encision; and Empatica’s wearable device for seizure detection in epilepsy patients.
- Ingrid Torjesen, Drug development: the journey of a medicine from lab to shelf (May 12, 2015), available at https://pharmaceutical-journal.com/article/feature/drug-development-the-journey-of-a-medicine-from-lab-to-shelf.
- E. Hope Weissler, et al., The role of machine learning in clinical research: transforming the future of evidence generation, Trials, 22:537 (2021).
- GAO Report, “Artificial Intelligence in Health Care – Benefits and Challenges of Machine Learning in Drug Development” (Dec. 2019), available at https://www.gao.gov/assets/gao-20-215sp.pdf.
- Google Products, Machine Learning - Advanced courses: GAN, available at https://developers.google.com/machine-learning/gan/problems.
- Varnavas D. Mouchlis, et al., Advances in De Novo Drug Design: From Convention to Machine Learning Methods
- Puru Rattan, et al., Artificial Intelligence and Machine Learning: What You Always Wanted to Know but Were Afraid to Ask, Gastro. Hep. Advances 2022; 1:70-78., available at https://reader.elsevier.com/reader/sd/pii/S277257232100025X?token=B19D6E40FB1E77A1EAFA4F8213B2B54C5B2A5BB5D16204633F16D80066D5514A7BE
6E6926F4E5B81FA354C3C6C3B46CF&originRegion=us-east-1&originCreation=20220921190222. - Karttikeya Mangalam, et al. Overcoming Mode Collapse with Adaptive Multi Adversarial Training (Dec. 2021), available at https://www.researchgate.net/publication/357417827_Overcoming_Mode_Collapse_with
_Adaptive_Multi_Adversarial_Training.
About The Author:
Tina Hu-Rodgers is counsel in Buchanan Ingersoll & Rooney’s Life Sciences industry group. She focuses her practice on issues related to the approval, regulation, promotion, sale, and reimbursement of drugs, medical devices, biologics, dietary supplements, foods, and cannabis-related products.