AI Is Unearthing New Drug Candidates, But It Still Needs Human Oversight
By Stephanie Rosner and Junaid Bajwa, DIA
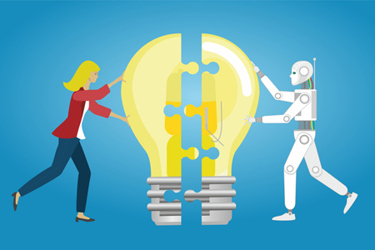
Drug discovery and development are complex, expensive, and time-consuming undertakings fraught with challenges at every stage, from identifying novel targets to navigating the regulatory process. However, recent advancements in artificial intelligence (AI) offer the potential to streamline and optimize drug development in unprecedented ways.
AI can accelerate timetables and minimize wasted resources by analyzing vast amounts of data from multiple sources, such as genomics, proteomics, metabolomics, and transcriptomics, to identify correlations that may not be immediately apparent to human researchers. It can model how drug molecules interact with biological targets in silico to avoid exponential costs, and it can more effectively pinpoint biomarkers that indicate how a disease progresses or responds to treatment, among other applications.
Bringing AI into this setting responsibly and ethically still requires adapting approaches and policies across an industry that's historically resistant to new methods. Still, there's a clear, demonstrated need to hasten the drug development process and get treatments in patients' hands sooner.
How Is AI Accelerating The Drug Discovery Process?
Ever since Exscienta announced in 2020 that a drug molecule designed by AI was set to enter human clinical trials, we’re seeing more examples of AI speeding up the drug discovery process.
At Novartis, scientists have used AI to create custom-designed molecules that target the root causes of diseases that have long eluded conventional treatments. Researchers can quickly scour data from thousands of past drug development experiments and use models to predict the most promising molecular structures, drastically shortening a timeline that can span years.
Novo Nordisk has taken a similar approach by using AI to summarize past trials and use what it learns to unravel the complexities of atherosclerosis, a leading cause of heart attack and stroke. It then incorporates additional AI models to identify novel targets and biomarkers, with the hope of successfully replicating this method to treat other diseases.
But targets aren’t just being identified or validated. They’re advancing through the development process. A survey of 20 AI-powered pharmaceutical companies led by the Boston Consulting Group in 2022 found that their drug candidates move from discovery to clinical trials at a breathtaking pace. These companies had 158 drug candidates at the discovery and preclinical stage at the time of the study, compared to 333 at the world’s 20 largest pharmaceutical companies. As the study noted, that’s “a combined pipeline equivalent to 50% of the in-house discovery and preclinical output of ‘Big Pharma.’”
The potential cost savings achieved through deploying AI in drug discovery are just as impressive as the faster timelines. A Wellcome report estimates that AI-driven research and development could slash costs by 25% to 50%, enabling biopharmaceutical companies to conceivably redirect those funds toward additional research that leads to a more robust drug discovery pipeline.
Of course, we must not forget that for all this to occur, one crucial element cannot be overlooked — human intervention.
Why We Must Ensure A Human Touch
While AI can automate countless tasks across the drug development ecosystem, it is not a replacement for human expertise.
AI tools must always have a "human in the loop" to perform as intended. That person, or ideally people, should not be passive, either. Humans should be active participants and critical evaluators who provide input and feedback, challenge and question the process, learn from it, and improve it. We also need to ensure the human is empowered and supported by the AI system, not replaced or undermined by it, and that the human is accountable and responsible for the outcomes and impacts of the AI system, not absolved or blamed by it.
We must maintain human oversight in drug discovery to address the limitations and biases of these AI systems. For example, AI has been known to experience "hallucinations," which occur when it generates false or nonsensical information because of a misinterpretation of its training data. In text-based outputs, this leads to inaccurate information, illogical statements, or fictional events being presented as truth. But when it comes to validating targets, AI may propose compounds that are chemically impossible to synthesize. It may also incorrectly identify targets as promising or dismiss valid targets due to errors in the data or algorithms. Humans must use their expertise to guide AI, incorporating rules and constraints that ensure the generated molecules are feasible and pragmatic.
Another critical reason for human oversight is the iterative nature of the drug discovery process. AI can generate promising drug candidates, but these compounds must be rigorously tested and refined. The results of these experiments provide valuable feedback that can be used to update and improve the AI models, creating a cycle of learning and optimization. Human researchers are essential in designing and conducting these experiments, interpreting the results, and using their domain expertise to refine the AI systems.
The success of AI in drug discovery will depend on our ability to strike the right balance between leveraging the power of these technologies and maintaining the essential role of human expertise. And that's important, given the level of scrutiny that AI-developed drugs will encounter before they reach the patients they're designed to treat.
Reaching Standards Spurs Confidence
Ultimately, what's crucial to effectively using AI in target identification and validation — and across the drug development process — is gaining regulatory acceptance.
Regulators are responsible for establishing and enforcing AI standards, ensuring the safety and efficacy of AI-enabled products and services, and protecting the rights and interests of patients and consumers. They are also the gatekeepers who will build public trust and confidence in any drug developed through AI-driven approaches.
But before a potential treatment can even be reviewed by a regulator, the biopharmaceutical company must have AI guidelines and best practices in place. This policy may rely in part on guidance offered by the U.S. Food and Drug Administration (FDA), the Organisation for Economic Co-operation and Development (OECD), the European Union (EU), or the White House, but it's imperative to remain abreast of any changes made by these institutions because all guidelines continue evolving.
You must first define and classify which tools and processes qualify as "AI." Then, your vision and strategy must align with the responsible AI principles of fairness, reliability, safety, privacy, security, inclusiveness, transparency, and accountability. You must also provide the necessary resources and infrastructure while fostering a culture of collaboration and innovation.
Likewise, your research team must select and apply the appropriate AI methods and tools, perhaps by turning to the OECD framework for guidance, then ensure the quality and integrity of the data and models before validating and interpreting the results. Regulators will want to see that appropriate procedures were followed when developing a drug candidate.
One of the primary obstacles to making all this happen is proper change management. It's no secret that the biopharmaceutical industry has historically been resistant to new methods, and incorporating AI tools requires a significant shift in mindset, workflows, and policies that it may not be prepared to handle. We must adopt a holistic and agile approach, as well as a culture of innovation and learning, to maximize AI's impact.
To overcome this, all stakeholders, including industry, regulators, patients, providers, payers, and policymakers, need to collaborate and communicate. By sharing their best practices and lessons learned from AI, and monitoring and evaluating the performance and impact of AI on an ongoing basis, everyone can create an environment that fosters responsible innovation.
If this doesn't happen, we could end up with scattershot, inefficient processes that don't justify the return on investment (ROI) in AI technologies. Companies may then be hesitant to invest further in AI, hindering its adoption and minimizing any chance it has of developing novel life-saving treatments. The failure rate, estimated at 90%, will remain high.
AI-driven drug discovery can significantly reduce costs and expedite the development process, potentially making drugs more accessible and addressing conditions that don't yet have a cure. It may even help us not just tackle diseases that affect the many but also those that affect the few. But its success will depend on the ability of all stakeholders to work together to balance innovation with regulation, ensuring patients, the public, and society benefit from these incredible innovations.
About the Authors:
Stephanie Rosner is a global AI associate at DIA, where she is dedicated to fostering ethical AI design and advancing technology with a human-centric approach. Rosner has held project management and business development roles at Mathematica Policy Research and Optum, working with stakeholders to ensure ethical and equitable outcomes and policies related to advancements in health projects.
Junaid Bajwa serves as a board member of DIA Global and is the chief medical scientist at Microsoft Research. He continues to practice as a physician in the U.K.’s National Health Service.